Open Access
ARTICLE
Multi-Classification Network for Identifying COVID-19 Cases Using Deep Convolutional Neural Networks
School of Computer and Software, Nanjing University of Information Science & Technology, Nanjing, 210044, China
* Corresponding Author: Ling Tan. Email:
Journal on Internet of Things 2021, 3(2), 39-51. https://doi.org/10.32604/jiot.2021.014877
Received 07 January 2021; Accepted 11 April 2021; Issue published 15 July 2021
Abstract
The novel coronavirus 2019 (COVID-19) rapidly spreading around the world and turns into a pandemic situation, consequently, detecting the coronavirus (COVID-19) affected patients are now the most critical task for medical specialists. The deficiency of medical testing kits leading to huge complexity in detecting COVID-19 patients worldwide, resulting in the number of infected cases is expanding. Therefore, a significant study is necessary about detecting COVID-19 patients using an automated diagnosis method, which hinders the spreading of coronavirus. In this paper, the study suggests a Deep Convolutional Neural Network-based multi-classification framework (COVMCNet) using eight different pre-trained architectures such as VGG16, VGG19, ResNet50V2, DenseNet201, InceptionV3, MobileNet, InceptionResNetV2, Xception which are trained and tested on the X-ray images of COVID-19, Normal, Viral Pneumonia, and Bacterial Pneumonia. The results from 4-class (Normal vs. COVID-19 vs. Viral Pneumonia vs. Bacterial Pneumonia) demonstrated that the pre-trained model DenseNet201 provides the highest classification performance (accuracy: 92.54%, precision: 93.05%, recall: 92.81%, F1-score: 92.83%, specificity: 97.47%). Notably, the DenseNet201 (4-class classification) pre-trained model in the proposed COV-MCNet framework showed higher accuracy compared to the rest seven models. Important to mention that the proposed COV-MCNet model showed comparatively higher classification accuracy based on the small number of pre-processed datasets that specifies the designed system can produce superior results when more data become available. The proposed multi-classification network (COV-MCNet) significantly speeds up the existing radiology based method which will be helpful for the medical community and clinical specialists to early diagnosis the COVID-19 cases during this pandemic.Keywords
Cite This Article
Citations
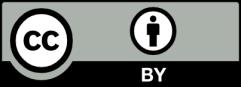