Open Access
ARTICLE
A Novel Integrated Machine & Business Intelligence Framework for Sensor Data Analysis
Vignan’s Institute of Engineering for Women, Andhra University, Visakhapatnam, India
* Corresponding Author: S. Kalyani. Email:
Journal on Internet of Things 2021, 3(1), 27-38. https://doi.org/DOI:10.32604/jiot.2021.013163
Received 12 October 2020; Accepted 07 December 2020; Issue published 16 March 2021
Abstract
Increased smart devices in various industries are creating numerous sensors in each of the equipment prompting the need for methods and models for sensor data. Current research proposes a systematic approach to analyze the data generated from sensors attached to industrial equipment. The methodology involves data cleaning, preprocessing, basics statistics, outlier, and anomaly detection. Present study presents the prediction of RUL by using various Machine Learning models like Regression, Polynomial Regression, Random Forest, Decision Tree, XG Boost. Hyper Parameter Optimization is performed to find the optimal parameters for each variable. In each of the model for RUL prediction RMSE, MAE are compared. Outcome of the RUL prediction should be useful for decision maker to drive the business decision; hence Binary cclassification is performed, and business case analysis is performed. Business case analysis includes the cost of maintenance and cost of non-maintaining a particular asset. Current research is aimed at integrating the machine intelligence and business intelligence so that the industrial operations optimized both in resource and profit.Keywords
Cite This Article
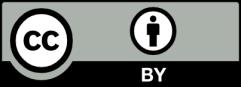
This work is licensed under a Creative Commons Attribution 4.0 International License , which permits unrestricted use, distribution, and reproduction in any medium, provided the original work is properly cited.