Open Access
ARTICLE
An Expected Patch Log Likelihood Denoising Method Based on Internal and External Image Similarity
School of Math and Statistics, Nanjing University of Information Science and Technology, Nanjing, 210044, China
* Corresponding Author: Jianwei Zhang. Email: .
Journal on Internet of Things 2020, 2(1), 13-21. https://doi.org/10.32604/jiot.2020.09073
Received 08 November 2019; Accepted 27 November 2019; Issue published 06 August 2020
Abstract
Nonlocal property is an important feature of natural images, which means that the patch matrix formed by similar image patches is low-rank. Meanwhile, learning good image priors is of great importance for image denoising. In this paper, we combine the image self-similarity with EPLL (Expected patch log likelihood) method, and propose an EPLL denoising model based on internal and external image similarity to improve the preservation of image details. The experiment results show that the validity of our method is proved from two aspects of visual and numerical results.Keywords
Cite This Article
Citations
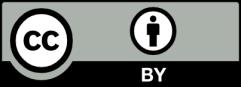