Open Access
ARTICLE
Intrusion Detection System Using a Distributed Ensemble Design Based Convolutional Neural Network in Fog Computing
1 Engineering Research Center of Intelligent Perception and Autonomous Control, Faculty of Information Technology, Beijing University of Technology, Beijing, 100124, China
2 Faculty of Computer Science and Engineering, GIK Institute of Engineering Sciences and Technology, Topi 23460, Pakistan
3 School of Engineering, Edith Cowan University, Joondalup Perth, WA 6027, Australia
* Corresponding Author: Shanshan Tu. Email:
Journal of Information Hiding and Privacy Protection 2022, 4(1), 25-39. https://doi.org/10.32604/jihpp.2022.029922
Received 15 March 2022; Accepted 05 May 2022; Issue published 17 June 2022
Abstract
With the rapid development of the Internet of Things (IoT), all kinds of data are increasing exponentially. Data storage and computing on cloud servers are increasingly restricted by hardware. This has prompted the development of fog computing. Fog computing is to place the calculation and storage of data at the edge of the network, so that the entire Internet of Things system can run more efficiently. The main function of fog computing is to reduce the burden of cloud servers. By placing fog nodes in the IoT network, the data in the IoT devices can be transferred to the fog nodes for storage and calculation. Many of the information collected by IoT devices are malicious traffic, which contains a large number of malicious attacks. Because IoT devices do not have strong computing power and the ability to detect malicious traffic, we need to deploy a system to detect malicious attacks on the fog node. In response to this situation, we propose an intrusion detection system based on distributed ensemble design. The system mainly uses Convolutional Neural Network (CNN) as the first-level learner. In the second level, the random forest will finally classify the prediction results obtained in the first level. This paper uses the UNSW-NB15 dataset to evaluate the performance of the model. Experimental results show that the model has good detection performance for most attacks.Keywords
Cite This Article
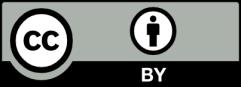
This work is licensed under a Creative Commons Attribution 4.0 International License , which permits unrestricted use, distribution, and reproduction in any medium, provided the original work is properly cited.