Open Access
ARTICLE
A Performance Study of Membership Inference Attacks on Different Machine Learning Algorithms
1
Department of Computer Science, University of Georgia, Athens, GA, 30602, USA
2
Department of Software Engineering, College of Computer Science and Engineering, University of Jeddah, Jeddah, 23890,
Saudi Arabia
* Corresponding Author: Mohammad Alahmadi. Email:
Journal of Information Hiding and Privacy Protection 2021, 3(4), 193-200. https://doi.org/10.32604/jihpp.2021.027871
Received 26 January 2022; Accepted 03 March 2022; Issue published 22 March 2022
Abstract
Nowadays, machine learning (ML) algorithms cannot succeed without the availability of an enormous amount of training data. The data could contain sensitive information, which needs to be protected. Membership inference attacks attempt to find out whether a target data point is used to train a certain ML model, which results in security and privacy implications. The leakage of membership information can vary from one machine-learning algorithm to another. In this paper, we conduct an empirical study to explore the performance of membership inference attacks against three different machine learning algorithms, namely, K-nearest neighbors, random forest, support vector machine, and logistic regression using three datasets. Our experiments revealed the best machine learning model that can be more immune to privacy attacks. Additionally, we examined the effects of such attacks when varying the dataset size. Based on our observations for the experimental results, we propose a defense mechanism that is less prone to privacy attacks and demonstrate its effectiveness through an empirical evaluation.Keywords
Cite This Article
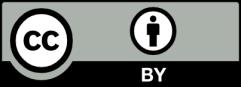