Open Access
ARTICLE
Verifiable Privacy-Preserving Neural Network on Encrypted Data
1
Nanjing University of Science and Technology, Nanjing, 210014, China
2
Jiangsu University, Zhenjiang, 212013, China
3
Nanyang Technological University, 639798, Singapore
* Corresponding Author: Chungen Xu. Email:
Journal of Information Hiding and Privacy Protection 2021, 3(4), 151-164. https://doi.org/10.32604/jihpp.2021.026944
Received 01 January 2022; Accepted 02 March 2022; Issue published 22 March 2022
Abstract
The widespread acceptance of machine learning, particularly of neural networks leads to great success in many areas, such as recommender systems, medical predictions, and recognition. It is becoming possible for any individual with a personal electronic device and Internet access to complete complex machine learning tasks using cloud servers. However, it must be taken into consideration that the data from clients may be exposed to cloud servers. Recent work to preserve data confidentiality has allowed for the outsourcing of services using homomorphic encryption schemes. But these architectures are based on honest but curious cloud servers, which are unable to tell whether cloud servers have completed the computation delegated to the cloud server. This paper proposes a verifiable neural network framework which focuses on solving the problem of data confidentiality and training integrity in machine learning. Specifically, we first leverage homomorphic encryption and extended diagonal packing method to realize a privacy-preserving neural network model efficiently, it enables the user training over encrypted data, thereby protecting the user’s private data. Then, considering the problem that malicious cloud servers are likely to return a wrong result for saving cost, we also integrate a training validation modular Proof-of-Learning, a strategy for verifying the correctness of computations performed during training. Moreover, we introduce practical byzantine fault tolerance to complete the verification progress without a verifiable center. Finally, we conduct a series of experiments to evaluate the performance of the proposed framework, the results show that our construction supports the verifiable training of PPNN based on HE without introducing much computational cost.Keywords
Cite This Article
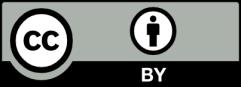
This work is licensed under a Creative Commons Attribution 4.0 International License , which permits unrestricted use, distribution, and reproduction in any medium, provided the original work is properly cited.