Open Access
ARTICLE
Adaptive Multi-Scale HyperNet with Bi-Direction Residual Attention Module for Scene Text Detection
College of Information Engineering, Shanghai Maritime University, Shanghai, China
* Corresponding Author: Jin Liu. Email:
Journal of Information Hiding and Privacy Protection 2021, 3(2), 83-89. https://doi.org/10.32604/jihpp.2021.017181
Received 23 January 2021; Accepted 29 March 2021; Issue published 30 July 2021
Abstract
Scene text detection is an important step in the scene text reading system. There are still two problems during the existing text detection methods: (1) The small receptive of the convolutional layer in text detection is not sufficiently sensitive to the target area in the image; (2) The deep receptive of the convolutional layer in text detection lose a lot of spatial feature information. Therefore, detecting scene text remains a challenging issue. In this work, we design an effective text detector named Adaptive Multi-Scale HyperNet (AMSHN) to improve texts detection performance. Specifically, AMSHN enhances the sensitivity of target semantics in shallow features with a new attention mechanism to strengthen the region of interest in the image and weaken the region of no interest. In addition, it reduces the loss of spatial feature by fusing features on multiple paths, which significantly improves the detection performance of text. Experimental results on the Robust Reading Challenge on Reading Chinese Text on Signboard (ReCTS) dataset show that the proposed method has achieved the state-of-the-art results, which proves the ability of our detector on both particularity and universality applications.Keywords
Cite This Article
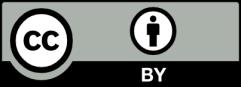
This work is licensed under a Creative Commons Attribution 4.0 International License , which permits unrestricted use, distribution, and reproduction in any medium, provided the original work is properly cited.