Open Access
REVIEW
Survey on the Loss Function of Deep Learning in Face Recognition
1 Director of Science and Technology Industry Department, Nanjing University of Information Science & Technology, Nanjing, China
2 School of Computer & Software, Jiangsu Engineering Center of Network Monitoring, Nanjing University of Information Science & Technology, Nanjing, China
3 Science and Technology Industry Department, Nanjing University of Information Science & Technology, Nanjing, China
4 Deptratment of Computer Science, King Saud University, Riyadh, Saudi Arabia
* Corresponding Author: Suncheng Feng. Email:
Journal of Information Hiding and Privacy Protection 2021, 3(1), 29-45. https://doi.org/10.32604/jihpp.2021.016835
Received 13 January 2021; Accepted 28 March 2021; Issue published 21 April 2021
Abstract
With the continuous development of face recognition network, the selection of loss function plays an increasingly important role in improving accuracy. The loss function of face recognition network needs to minimize the intra-class distance while expanding the inter-class distance. So far, one of our mainstream loss function optimization methods is to add penalty terms, such as orthogonal loss, to further constrain the original loss function. The other is to optimize using the loss based on angular/cosine margin. The last is Triplet loss and a new type of joint optimization based on HST Loss and ACT Loss. In this paper, based on the three methods with good practical performance and the joint optimization method, various loss functions are thoroughly reviewed.Keywords
Cite This Article
Citations
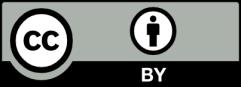
This work is licensed under a Creative Commons Attribution 4.0 International License , which permits unrestricted use, distribution, and reproduction in any medium, provided the original work is properly cited.