Open Access
ARTICLE
Random Forests Algorithm Based Duplicate Detection in On-Site Programming Big Data Environment
1 University of Science and Technology Beijing, Beijing, 100083, China
2 Beijing University of Posts and Telecommunications, Beijing, 100876, China
3 Systems Engineering Institute AMS, Beijing, 100071, China
4 Audio Analytic, 2 Quayside, Cambridge, CB5 8AB, UK
* Corresponding Author: Lei Guo. Email:
Journal of Information Hiding and Privacy Protection 2020, 2(4), 199-205. https://doi.org/10.32604/jihpp.2020.016299
Received 18 December 2020; Accepted 02 January 2021; Issue published 07 January 2021
Abstract
On-site programming big data refers to the massive data generated in the process of software development with the characteristics of real-time, complexity and high-difficulty for processing. Therefore, data cleaning is essential for on-site programming big data. Duplicate data detection is an important step in data cleaning, which can save storage resources and enhance data consistency. Due to the insufficiency in traditional Sorted Neighborhood Method (SNM) and the difficulty of high-dimensional data detection, an optimized algorithm based on random forests with the dynamic and adaptive window size is proposed. The efficiency of the algorithm can be elevated by improving the method of the keyselection, reducing dimension of data set and using an adaptive variable size sliding window. Experimental results show that the improved SNM algorithm exhibits better performance and achieve higher accuracy.Keywords
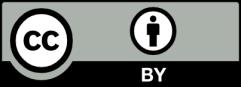