Open Access
ARTICLE
A Location Prediction Method Based on GA-LSTM Networks and Associated Movement Behavior Information
1 Hunan University of Science and Technology, Xiangtan, 411201, China
2 Deakin University, Geelong, 3216, Australia
* Corresponding Author: Liming Jiang. Email:
Journal of Information Hiding and Privacy Protection 2020, 2(4), 187-197. https://doi.org/10.32604/jihpp.2020.016243
Received 20 December 2020; Accepted 01 January 2021; Issue published 07 January 2021
Abstract
Due to the lack of consideration of movement behavior information other than time and location perception in current location prediction methods, the movement characteristics of trajectory data cannot be well expressed, which in turn affects the accuracy of the prediction results. First, a new trajectory data expression method by associating the movement behavior information is given. The pre-association method is used to model the movement behavior information according to the individual movement behavior features and the group movement behavior features extracted from the trajectory sequence and the region. The movement behavior features based on pre-association may not always be the best for the prediction model. Therefore, through association analysis and importance analysis, the final association feature is selected from the pre-association features. The trajectory data is input into the LSTM networks after associated features and genetic algorithm (GA) is used to optimize the combination of the length of time window and the number of hidden layer nodes. The experimental results show that compared with the original trajectory data, the trajectory data associated with the movement behavior information helps to improve the accuracy of location prediction.Keywords
Cite This Article
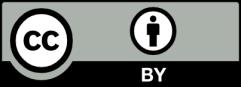
This work is licensed under a Creative Commons Attribution 4.0 International License , which permits unrestricted use, distribution, and reproduction in any medium, provided the original work is properly cited.