Open Access
REVIEW
A Survey on Recent Advances in Privacy Preserving Deep Learning
1 Nanjing University of Information Science & Technology, Nanjing, 210044, China
2 Engineering Research Center of Digital Forensics, Ministry of Education, China
* Corresponding Author: Leiming Yan. Email:
Journal of Information Hiding and Privacy Protection 2020, 2(4), 175-185. https://doi.org/10.32604/jihpp.2020.010780
Received 13 August 2020; Accepted 01 October 2020; Issue published 07 January 2021
Abstract
Deep learning based on neural networks has made new progress in a wide variety of domain, however, it is lack of protection for sensitive information. The large amount of data used for training is easy to cause leakage of private information, thus the attacker can easily restore input through the representation of latent natural language. The privacy preserving deep learning aims to solve the above problems. In this paper, first, we introduce how to reduce training samples in order to reduce the amount of sensitive information, and then describe how to unbiasedly represent the data with respect to specific attributes, clarify the research results of other directions of privacy protection and its corresponding algorithms, summarize the common thoughts and existing problems. Finally, the commonly used datasets in the privacy protection research are discussed in this paper.Keywords
Cite This Article
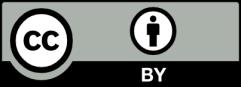