Open Access
REVIEW
A Survey on Adversarial Example
Nanjing University of Information Science and Technology, Nanjing, 210044, China
* Corresponding Author: Jiawei Zhang. Email:
Journal of Information Hiding and Privacy Protection 2020, 2(1), 47-57. https://doi.org/10.32604/jihpp.2020.010462
Received 20 May 2020; Accepted 01 July 2020; Issue published 15 October 2020
Abstract
In recent years, deep learning has become a hotspot and core method in the field of machine learning. In the field of machine vision, deep learning has excellent performance in feature extraction and feature representation, making it widely used in directions such as self-driving cars and face recognition. Although deep learning can solve large-scale complex problems very well, the latest research shows that the deep learning network model is very vulnerable to the adversarial attack. Add a weak perturbation to the original input will lead to the wrong output of the neural network, but for the human eye, the difference between origin images and disturbed images is hardly to be notice. In this paper, we summarize the research of adversarial examples in the field of image processing. Firstly, we introduce the background and representative models of deep learning, then introduce the main methods of the generation of adversarial examples and how to defend against adversarial attack, finally, we put forward some thoughts and future prospects for adversarial examples.Keywords
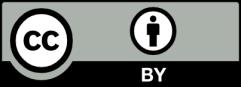