Open Access
ARTICLE
Research on Denoising of Cryo-em Images Based on Deep Learning
Key Laboratory of Intelligent Computing & Information Processing, Ministry of Education, Xiangtan University, Xiangtan, 411105, China
* Corresponding Author: Jianquan Ouyang. Email:
Journal of Information Hiding and Privacy Protection 2020, 2(1), 1-9. https://doi.org/10.32604/jihpp.2020.010657
Received 15 May 2020; Accepted 30 May 2020; Issue published 15 October 2020
Abstract
Cryo-em (Cryogenic electron microscopy) is a technology this can build bio-macromolecule of three-dimensional structure. Under the condition of now, the projection image of the biological macromolecule which is collected by the Cryo-em technology that the contrast is low, the signal to noise is low, image blurring, and not easy to distinguish single particle from background, the corresponding processing technology is lagging behind. Therefore, make Cryoem image denoising useful, and maintaining bio-macromolecule of contour or signal of function-construct improve Cryo-em image quality or resolution of Cryo-em three-dimensional structure have important effect. This paper researched a denoising function base on GANs (generative adversarial networks), purpose an improved discriminant model base on Wasserstein distance and an improved image denoising model by add gray constraint. Our model turn discriminant model’s training process from binary classification’s training process into regression task training process, it make GANs in training process more stable, more reasonable parameter passing. Meantime, we also propose an improved generative model by add gray constraint. The experimental results show that our model can increase the peak signal-to-noise ratio of the Cryo-em simulation image by 10.3 dB and improve SSIM (Structural Similarity Index) of the denoised image results by 0.43. Compared with traditional image denoising algorithms such as BM3D (Block Matching 3D), our model can better save the model structure and the vein signal in the original image and the operation speed is faster.Keywords
Cite This Article
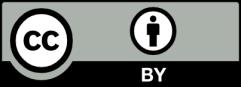
This work is licensed under a Creative Commons Attribution 4.0 International License , which permits unrestricted use, distribution, and reproduction in any medium, provided the original work is properly cited.