Open Access
ARTICLE
Deep Learning Trackers Review and Challenge
School of Computer and Software, Nanjing University of Information Science and Technology, 210044, China.
School of Information Science and Engineering, Southeast University, 210096, China.
State Key Laboratory for Novel Software Technology, Nanjing University, China.
The Center for Machine Vision and Signal Analysis, University of Oulu, FI-90014 Oulu, Finland.
*Corresponding Author: Xu Cheng. Email: .
Journal of Information Hiding and Privacy Protection 2019, 1(1), 23-33. https://doi.org/10.32604/jihpp.2019.05938
Abstract
Recently, deep learning has achieved great success in visual tracking. The goal of this paper is to review the state-of-the-art tracking methods based on deep learning. First, we categorize the existing deep learning based trackers into three classes according to network structure, network function and network training. For each categorize, we analyze papers in different categories. Then, we conduct extensive experiments to compare the representative methods on the popular OTB-100, TC-128 and VOT2015 benchmarks. Based on our observations. We conclude that: (1) The usage of the convolutional neural network (CNN) model could significantly improve the tracking performance. (2) The trackers with deep features perform much better than those with low-level hand-crafted features. (3) Deep features from different convolutional layers have different characteristics and the effective combination of them usually results in a more robust tracker. (4) The deep visual trackers using end-to-end networks usually perform better than the trackers merely using feature extraction networks. (5) For visual tracking, the most suitable network training method is to per-train networks with video information and online fine-tune them with subsequent observations. Finally, we summarize our manuscript and highlight our insights, and point out the further trends for deep visual tracking.Keywords
Cite This Article
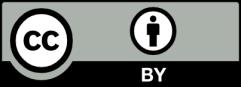