Open Access
ARTICLE
Hybrid Model for Short-Term Passenger Flow Prediction in Rail Transit
1 China Research Center for Emergency Management, Wuhan University of Technology, Wuhan, 430070, China
2 Hubei Provincial Crisis and Disaster Emergency Management Research Center, Wuhan, 430070, China
* Corresponding Author: Wei Zhang. Email:
Journal on Big Data 2023, 5, 19-40. https://doi.org/10.32604/jbd.2023.038249
Received 04 December 2022; Accepted 10 April 2023; Issue published 05 October 2023
Abstract
A precise and timely forecast of short-term rail transit passenger flow provides data support for traffic management and operation, assisting rail operators in efficiently allocating resources and timely relieving pressure on passenger safety and operation. First, the passenger flow sequence models in the study are broken down using VMD for noise reduction. The objective environment features are then added to the characteristic factors that affect the passenger flow. The target station serves as an additional spatial feature and is mined concurrently using the KNN algorithm. It is shown that the hybrid model VMD-CLSMT has a higher prediction accuracy, by setting BP, CNN, and LSTM reference experiments. All models’ second order prediction effects are superior to their first order effects, showing that the residual network can significantly raise model prediction accuracy. Additionally, it confirms the efficacy of supplementary and objective environmental features.Keywords
Cite This Article
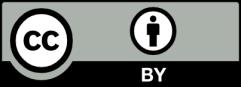
This work is licensed under a Creative Commons Attribution 4.0 International License , which permits unrestricted use, distribution, and reproduction in any medium, provided the original work is properly cited.