Open Access
ARTICLE
Social Opinion Network Analytics in Community Based Customer Churn Prediction
1 Country bowen University, Iwo, +234, Nigeria
2 University of Ibadan, Ibadan, +234, Nigeria
* Corresponding Author: Ayodeji O. J Ibitoye. Email:
Journal on Big Data 2022, 4(2), 87-95. https://doi.org/10.32604/jbd.2022.024533
Received 23 October 2021; Accepted 27 June 2022; Issue published 31 October 2022
Abstract
Community based churn prediction, or the assignment of recognising the influence of a customer’s community in churn prediction has become an important concern for firms in many different industries. While churn prediction until recent times have focused only on transactional dataset (targeted approach), the untargeted approach through product advisement, digital marketing and expressions in customer’s opinion on the social media like Twitter, have not been fully harnessed. Although this data source has become an important influencing factor with lasting impact on churn management. Since Social Network Analysis (SNA) has become a blended approach for churn prediction and management in modern era, customers residing online predominantly and collectively decide and determines the momentum of churn prediction, retention and decision support. In existing SNA approaches, customers are classified as churner or non-churner (1 or 0). Oftentimes, the customer’s opinion is also neglected and the network structure of community members are not exploited. Consequently, the pattern and influential abilities of customers’ opinion on relative members of the community are not analysed. Thus, the research developed a Churn Service Information Graph (CSIG) to define a quadruple churn category (churner, potential churner, inertia customer, premium customer) for non-opinionated customers via the power of relative affinity around opinionated customers on a direct node to node SNA. The essence is to use data mining technique to investigate the patterns of opinion between people in a network or group. Consequently, every member of the online social network community is dynamically classified into a churn category for an improved targeted customer acquisition, retention and/or decision supports in churn management.Keywords
In today’s business world, the rapid growth in the use of social media has made SNA an improved parameter with enhanced predictive power for customer prediction and retention models. While churn prediction is the ability of a customer to stops contract with one company in order to become a customer of another, Social Network (SN) is a set of people or groups of people with interactions between them and/or some pattern of contacts [1]. Consequently, SNA is a set of research procedures for identifying group of people who shared common structures in systems based on the existing relationship among actors. SNA consists of several related works such as alpha users within the communities, community detection, hub member and non-hub members’ identification [2] among others.
In churn prediction, whenever a customer stops the contract with one company to become a customer of a competitor, the customer is considered as lost or churn. Significantly, all churn acts do not happen suddenly, oftentimes, it is a process that is nurtured consistently in a conducive environment until desired remedy is no longer satisfactory to a customer. Thus, with endless reasons for customer churn, sometimes, customers may not announce their decision to quit a service provider, however, intrinsic expression about an organization’s brand and its community impact are factors, which require timely analysis in order to predict the churn class of a customer [3]. Although, conventional approaches for churn prediction as largely focused on various learning models. These processes treat individual customer’s independently through the feature-based modelling techniques. However, it lacked power to analyse the role of social ties between individuals while determining the propensity to churn.
In digitised social community, social tie is necessary for effective decision support to a customer and to the community members of the respective customer. While [4] developed a fuzzified support vector approach to identify the bias in text, it also reclassified opinions into different degrees of positive and negative class through fuzzy membership extension on a support vector model so as to accommodate inherent ambiguity in spoken words. In addition, several other existing churn classification models via social network analysis directly mine opinions into strict positive and negative categories, the obtained values through this approach tentatively defines the churn category of a customer with positive opinion as non-churner, with negative opinion equivalent to churner. These approaches do not consider the network structure through which other members of the community can be classified. Thus, the power of communal influence via relative affinity is neglected while targeted decision support for customer acquisition and retention becomes more difficult for organizations to manage. With this, organisations spend five-six times to acquire a new customer than retaining an existing one.
Therefore, this research developed a CSIG for community-based churn classification via direct node to node analysis of relative strength and influence capacity of an opinionated user to non-opinionated member in a community. The CSIG is a network structure of customer with direct and indirect links. Here, the relationship affinity through degree and closeness centralities uses customer’s network strength to identify patterns and impact of an opinionate customer on the immediate non-opinionated community members. It also measures the communication throughput and influence among community members. Thus, the probability of influencing a non-opinionate customer by an opinionated one, alongside the connection strength between the dual as members of the same SN community are obtained. The essence is to maximize the potency in community members’ freedom of expression while as members remained connected in short chains of intermediate friends; over a period, the evolution and analytics of SN continues to spread rapidly. Also, as new friendships is constantly formed through random encounters, more patterns for churn decision support are obtained. Therefore, by introducing mutual friends, or further association through common interests and opinion the CSIG application becomes more robust for consumers’ behavioural management. Hence, in Section 2, an overview of churn prediction strategies in CRM with SNA influence in communitybased churn prediction is discussed. The developed CSIG is presented in Section 3 while sample experiment and result evaluations are discussed in Section 4. Later, proposed future work is presented in Section 5 before the conclusion is documented in Section 6.
2 Related Works in SNA and Churn Prediction Strategies in CRM
For many decades, understanding why customers terminate relationships has been a focus of marketing research [5]. The strategic approach has been through customer relationship management (CRM), which targets the development of profitable, long-term relationships with customers and stakeholders [6]. Basically, CRM are operational or analytical driven. While operational CRM deals with collecting customer data through a range of touch points such as contact management system, the analytical tools are adopted to analyse customer data that are stored in databases for the purpose of profiling, segmentations and also pattern generation from customer behaviours in order to predict the churn class of a customer [7].
In more recent times, effective usage of CRM has drifted towards customer centric, targeted marketing instead of the long existing product centric, and mass marketing strategies. However, utilizing this comes with prominent issues due to the competitive nature of service-based industries; such as telecommunication, banking, and online gaming in churn prediction [8]. With the introduction of social networking as a new era of content creation where individuals can easily share experiences and information with other users [9], the development as also enabled customers and businesses to collaborate on the internet. Thus, by using social media, consumers can create content and offer valuable advice to others. When people share their reviews, information, advice, warnings, tips and/or any kind of issues that are interesting to their “connection”, the information becomes a helpful source, which may influence consumer’s decision-making to a significant degree [10]. This is achieved through the impact of social network analysis.in churn prediction.
Therefore, with groups of people who do not share or that shares similar interests, backgrounds and activities but essentially bound to build social networks via the social media, such as microblogs e.g., Twitter has provided interactive and cheaper way for user to share ideas, exchange information and stay connected with people [11]. This group of people share and upload contents with images, texts and videos. They communicate with each other, and the power of influence is demonstrated among member. Thus, a SN structure is constituted by a set of ‘nodes’ connected with different ‘ties’ [12]. The cardinality of nodes expands dynamically, especially on the web, as new nodes and profiles are created continuously, while further populating the social web [13]. Hence, network thinking has contributed a number of important insights about social power while the different network analysis approach emphasize that power is inherently relational. When there are common set of connections, the social scientists call these connections ‘network’ [14].
In addition, a SN consist of a set of individuals and some form of social relationship that ties the individuals together [15]. However, SNA is the analysis of social communication through network and graph theory [16]. It models and visualize social networks in order to detect social structures. In telecommunication, among others, SNA has been explored through customer data and Call Detail Data (CDR). For instance, [17] used detailed CDR of mobile operator to build a connection graph between subscribers; based on calls made between members while [18] predicted customer churn through interpersonal influence using SNA. Just as churn prediction model was modelled for multi-players in online games via the use of the spreading activation [19]. Reference [20], also developed a churn model in telecommunication sector as a spread of influence by also applying the spreading activation method. Among others, [21] used social groups to predict customer churn in mobile networks. However, to effectively manage SNA community with dynamic capacities to expand, in Section 4, the developed CSIG is discussed. Here, pattern of customer’s influence and impact of each opinionated customer in SNA towards churn management of customers using a newly defined quadruple churn categories against existing binary churn prediction model are discussed.
3 Developed Churn Service Information Graph
One distinct attribute of a social community is that not every member exhibits the same traits. For instance, some members’ freely expressed opinion frequently, while some are passive. Despite these differences, by words of expression, community members can be influenced to a significant degree. Thus, in recent times, a community of many customers with relative association have become more universal through the power of the social media. Now, families, friends, colleagues, etc. communicate more often on the social media due to several factors like work, location, and physical distancing. This factors among others, inhibits physical social communication among friends and loved once. Consequently, as people edit, like, follow, link, reply, retweet, make comment, tag, review, and update information on their Twitter account, they form collections of connections. Thus, CSIG uses the analyses of people’s opinion through “word of mouth” and the influence of such on other people’s decision in SN community and among overlapping communities. Here, the SN influence is based on various degree of centrality with special emphasis on users’ opinion. By this, influence degree is not only about centrality measurement of the most influential person in the network; meaning, a person is not only considered to be the most influential based on connected nodes in the network but also by direct/indirect impact of users’ opinion analysis though Degree Centrality, and Closeness Centrality.
Here, the degree centrality was used to ascertain the total number of targeted or dependent nodes the expressed opinion of the source node can influence. Likewise, closeness centrality measures how fast can the source node expressed opinion reach the dependent or targeted node in the network. By these two centrality measures, a value is obtained to showcase the connection of the source node to other well connected dependent node in the network. Therefore, through the community of online social networker, we define and represent two distinct nodes as following:
Definition 1: An opinionated (active user) node as a social user who has expressed, is expressing or may express opinion(s) about a product or brand of an organization over a period.
Definition 2: A non-opinionated (passive user) node as a social user who does not express, is not willing to express and/or may not express opinion(s) about a product or brand of an organization over a period.
The definition 1 and 2 is further illustrated in the Fig. 1. PU are passive users and AU are the Active users. The bi-directional arrow heads, signifies an existence of two-way communication between nodes while the directed edges indicated that one node is following the other.
Figure 1: Churn service information graph
By analyzing the social network graph using algorithm listing 1, the communication weight between nodes, and degree of influence between nodes on the social network graph is generated. Here, if an influential node in a community is a churner, the probability that dependent nodes around such can also churn becomes significant and is given necessary consideration. Hence, the CSIG defines the churn class of other members of the community around an active node for churn classification. The objective is to measure the relative degree of affinity between the passive nodes and the active nodes in the social community using the algorithm in listing 1 for effective decision support in churn management.
From algorithm listing 1, churn classes were computed for every member of the community where relative scores were obtained for each node. While the scores are not static, it changes based on node strength and affiliation over a period
By these algorithms, the churn classification of non-opinionated customers around opinioned once is predominantly based on pattern analysis and influence of node through customer’s communication weight score, which exist between nodes on a social network. The algorithm listing 1, measures the total amount of direct links an opinionated node has with the other nodes around it on the network while algorithm listing 2, classifies each customer according to the score obtained via the analysis. The essence of the new classification threshold (Premium Customer, Inertia Customer, Potential Churner, and Churner) is triggered on the fact that to a certain degree every customer is a potential churner. Thus, identifying a customer as churner or non-churner leads to churn misclassification with unreliable decision support system for churn management. In section four, same experiments based of twitter feeds and network structure is presented.
Strong positive = Premium customer
Positive = Inertia customer
Negative = Potential churner
Strong negative = Churner
There, to evaluate the CSIG model, we presented a sample of customer across different churn class and the following results as obtained are presented;
The experiment is an analysis of 32201 opinioned Twitter users with different network structure and connections. The clustered network represented users following a telecoms organization with at least an opinion that contains the organization’s keyword, product brand, services, promotions and hashtags. The opinions of the users are mined with each belonging to one of the four-classed churn category as earlier defined. The opinion class represented a churn category wherein every member of the community fit into for real time churn analysis. Thus, this opinion mining class are further represented as follows.
Thus, the evaluation report for each of the customer in Table 1 is presented in Tables 2–5 respectively.
By this impact ratio of churn classification with the sampled customer records, a new cluster of churn class were achieved among non-opinionated members of the community. By this, organizations can effectively target subsets of a community cluster with recommendations to different categories of premium customer, inertia customer, potential churner and churner.
While the current research work is based on direct node to node connection in a community, in the nearest future, we hope to focus on overlapping communities and its impact on churn prediction in community-based churn management. Thereby, evaluating the achieved result with the CSIG model presented here with others.
With the continuous growth in digital marketing and it influence on customer relationship management, here, the research approached churn management from a social network opinion mining perspective. Although, existing approach performs SNA on customer data records, here customers’ opinion is processed to determine their churn class. Later, the churn classes of community members around active customers are mined for a more effective targeted decision support. The algorithm, sample experiments and evaluations are presented earlier showed that churn classification via opinion mining is no longer restricted to opinionated customers but every community member on a social network.
Funding Statement: The authors received no specific funding for this study.
Conflicts of Interest: The authors declare that they have no conflicts of interest to report regarding the present study.
References
1. M. E. J. Newman, “The mathematics of networks,” 2007. [Google Scholar]
2. S. Wu, L. Y. Wu, H. Y. Jin and S. J. Gu, “Customer ranking authority-hub algorithm for mobile communications,” in Fourth Int. Conf. on Fuzzy Systems and Knowledge Discovery, Haikou, China, pp. 559–563, 2007. [Google Scholar]
3. W. Verbeke, K. Dejaeger, D. Martens, J. Hur and B. Baesens, “New insights into churn prediction in the telecommunication sector: A profit driven data mining approach,” European Journal of Operational Research, vol. 218, no. 1, pp. 211–229, 2011. [Google Scholar]
4. A. O. J. Ibitoye and O. F. W. Onifade, “Making sense out of social data: A generic fuzzified support vector approach (FSVA) for public opinion classification on telecoms data,” in IEEE-EECCMC Conf. September 8 & 9, GRT Institute of Engineering and Technology, India, 2018. [Google Scholar]
5. D. Jain and S. S. Singh, “Customer lifetime value research in marketing: A review and future directions,” Journal of Interactive Marketing, vol. 16, no. 2, pp. 34–46, 2002. [Google Scholar]
6. A. Payne and P. Frow, “A strategic framework for customer relationship management,” Journal of Marketing, vol. 69, no. 4, pp. 17–167, 2005. [Google Scholar]
7. G. Shmueli and O. R. Koppius, “Predictive analytics in information systems research,” MIS Quarterly, pp. 289–296, 2011. [Google Scholar]
8. J. Lang and F. S. Wu, “Social network user lifetime,” in IEEE/ACM Int. Conf. on Advances in Social Networks Analysis and Mining, Kaohsiung, Taiwan, 2011. [Google Scholar]
9. L. Xi, W. Yin, A. Le, H. Ni, L. Huang et al., “Churn analysis of online social network users using data mining techniques,” Lecture Notes in Engineering & Computer Science, vol. 2195, no. 1, pp. 551–556, 2012. [Google Scholar]
10. L. P. Forbes and E. M. Vespoli, “Does social media influence consumer buying behavior? An investigation of recommendations and purchases,” Journal of Business & Economics Research, vol. 11, no. 2, pp. 107–111, 2013. [Google Scholar]
11. D. Boyd and N. Ellison, “Social network sites: Definition, history, and scholarship,” Journal of Computer-Mediated Communication, vol. 13, no. 1, pp. 210–230, 2007. [Google Scholar]
12. J. D. Luo, in Social Network Analysis, Beijing: Social Science Academic Press, 2010. [Google Scholar]
13. D. M. Ehrlich, “Social network survey paper,” International Journal of Learning and Intellectual Capital, vol. 3, no. 2, pp. 167–177, 2006. [Google Scholar]
14. A. Giddens, Sociology, Cambridge: Polity Press, 2009. [Google Scholar]
15. L. A. Adamic and E. Adar, “Friends and neighbors on the web,” Social Networks, vol. 25, no. 3, pp. 211–230, 2003. [Google Scholar]
16. A. D. Andrea, F. Ferri and P. Grifoni, “An overview of methods for virtual social network analysis,” Computational Social Network Analysis, pp. 3–25, 2009. https://doi.org/10.1007/978-1-84882-229-0_1. [Google Scholar]
17. K. Dasgupta, R. Singh, B. Viswanathan, D. Chakraborty, S. Mukherjea et al., “Social ties and their relevance to churn in mobile telecom networks,” in Int. Conf. on Extending Database Technology, New york, NY, USA, pp. 668–677, 2008. [Google Scholar]
18. X. Zhang, J. Zhu, S. Xu and Y. Wan, “Predicting customer churn through interpersonal influence,” Knowledge-Based Systems, vol. 28, pp. 97–104, 2012. [Google Scholar]
19. J. Kawale, A. Pal and J. Srivastava, “Churn prediction in MMORPGs: A social influence based approach,” Computational Science and Engineering, vol. 4, pp. 423–428, 2009. [Google Scholar]
20. K. Dasgupta, R. Singh and B. Viswanathan, “Social ties and their relevance to churn in mobile telecom networks,” in Int. Conf. on Edbt, New york, NY, USA, pp. 668–677, 2008. [Google Scholar]
21. Y. Richter, E. Yom-Tovy and N. Slonim, “Predicting customer churn in mobile networks through analysis of social groups,” in Siam Int. Conf. on Data Mining, Columbus, Ohio, USA, pp. 732–741, 2010. [Google Scholar]
Cite This Article
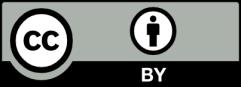
This work is licensed under a Creative Commons Attribution 4.0 International License , which permits unrestricted use, distribution, and reproduction in any medium, provided the original work is properly cited.