Open Access
ARTICLE
A Noise Extraction Method for Cryo-EM Single-Particle Denoising
1 Key Laboratory of Intelligent Computing Information Processing, Ministry of Education, School of Computer Science and School of Cyberspace Science, Xiangtan University, Xiangtan, 411100, China
2 Department of Computer Science, The University of Georgia, Georgia, 30301, USA
* Corresponding Author: Jianquan Ouyang. Email:
Journal on Big Data 2022, 4(1), 61-76. https://doi.org/10.32604/jbd.2022.028078
Received 04 March 2022; Accepted 05 April 2022; Issue published 04 May 2022
Abstract
Cryo-Electron Microscopy (cryo-EM) has become a powerful method to study the structure and function of biological macromolecules. However, in clustering tasks based on the projection angle of particles in cryo-EM, the noise considerably affects the clustering results. Existing denoising algorithms are ineffective due to the extremely low signal-to-noise ratio (SNR) of cryo-EM images and the complexity of noise types. The noise of a single particle greatly influences the orientation estimation of the subsequent clustering task, and the result of the clustering task directly affects the accuracy of the 3D reconstruction. In this paper, we propose a construction method of cryo-EM denoising dataset that uses U-Net to extract noise blocks from cryo-EM images, superimpose the noise block with the projected pure particles to construct our simulated dataset. Then we adopt a supervised generative adversarial network (GAN) with perceptual loss to train on our simulated dataset and denoise the real cryo-EM single particle. The method can solve the problem of poor denoising performance caused by assuming that the noise of the Gaussian distribution does not conform to the noise distribution of cryo-EM, and it can retain the useful information of particles to a great extent. We compared traditional image filtering methods and the classic deep learning denoising algorithm DnCNN on the simulated and real datasets. Experiment results show that the method based on deep learning has more advantages than traditional image denoising methods. It is worth mentioning that our method achieves a competitive peak signal to noise ratio (PSNR) and structural similarity (SSIM). Moreover, visualization results, indicate that our method can retain the structure information and orientation information of particles to a greater extent compared with other state-of-the-art image denoising methods. It means that our denoising task can provide considerable help for subsequent cryo-EM clustering tasks.Keywords
Cite This Article
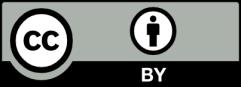
This work is licensed under a Creative Commons Attribution 4.0 International License , which permits unrestricted use, distribution, and reproduction in any medium, provided the original work is properly cited.