Open Access
ARTICLE
WMA: A Multi-Scale Self-Attention Feature Extraction Network Based on Weight Sharing for VQA
Shanghai Maritime University, Shanghai, 201306, China
* Corresponding Author: Jin Liu. Email:
Journal on Big Data 2021, 3(3), 111-118. https://doi.org/10.32604/jbd.2021.017169
Received 22 January 2021; Accepted 20 June 2021; Issue published 22 November 2021
Abstract
Visual Question Answering (VQA) has attracted extensive research focus and has become a hot topic in deep learning recently. The development of computer vision and natural language processing technology has contributed to the advancement of this research area. Key solutions to improve the performance of VQA system exist in feature extraction, multimodal fusion, and answer prediction modules. There exists an unsolved issue in the popular VQA image feature extraction module that extracts the fine-grained features from objects of different scale difficultly. In this paper, a novel feature extraction network that combines multi-scale convolution and self-attention branches to solve the above problem is designed. Our approach achieves the state-of-the-art performance of a single model on Pascal VOC 2012, VQA 1.0, and VQA 2.0 datasets.Keywords
Cite This Article
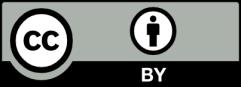
This work is licensed under a Creative Commons Attribution 4.0 International License , which permits unrestricted use, distribution, and reproduction in any medium, provided the original work is properly cited.