Open Access
ARTICLE
OPPR: An Outsourcing Privacy-Preserving JPEG Image Retrieval Scheme with Local Histograms in Cloud Environment
Nanjing University of Information Science & Technology, Nanjing, 210044, China
* Corresponding Author: Zhihua Xia. Email:
Journal on Big Data 2021, 3(1), 21-33. https://doi.org/10.32604/jbd.2021.015892
Received 10 September 2020; Accepted 20 December 2020; Issue published 25 January 2021
Abstract
As the wide application of imaging technology, the number of big image data which may containing private information is growing fast. Due to insufficient computing power and storage space for local server device, many people hand over these images to cloud servers for management. But actually, it is unsafe to store the images to the cloud, so encryption becomes a necessary step before uploading to reduce the risk of privacy leakage. However, it is not conducive to the efficient application of image, especially in the Content-Based Image Retrieval (CBIR) scheme. This paper proposes an outsourcing privacypreserving JPEG CBIR scheme. We design a set of JPEG format-compatible encryption method, making no file expansion to JPEG files. We firstly combine multiple adjacent 8 × 8 DCT coefficient blocks into big-blocks. Then, random scrambling and stream encryption are used on the binary code of DCT coefficients to protect the JPEG image privacy. The task of extracting features from encrypted images and retrieving similar images are done by the cloud server. The group index histograms of DCT coefficients are extracted from the encrypted big-blocks, then the global vector is produced to represent the JPEG image with the aid of bagof-words (BOW) model. The security analysis and experimental results show that our proposed scheme has strong security and good retrieval performance.Keywords
Cite This Article
Citations
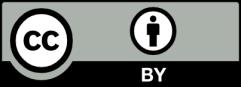
This work is licensed under a Creative Commons Attribution 4.0 International License , which permits unrestricted use, distribution, and reproduction in any medium, provided the original work is properly cited.