Open Access
ARTICLE
Multi-Scale Blind Image Quality Predictor Based on Pyramidal Convolution
School of Computer and Software, Nanjing University of Information Science and Technology, Nanjing, 210044, China
* Corresponding Author: Xiao Shao. Email:
Journal on Big Data 2020, 2(4), 167-176. https://doi.org/10.32604/jbd.2020.015357
Received 17 September 2020; Accepted 04 December 2020; Issue published 24 December 2020
Abstract
Traditional image quality assessment methods use the hand-crafted features to predict the image quality score, which cannot perform well in many scenes. Since deep learning promotes the development of many computer vision tasks, many IQA methods start to utilize the deep convolutional neural networks (CNN) for IQA task. In this paper, a CNN-based multi-scale blind image quality predictor is proposed to extract more effectivity multi-scale distortion features through the pyramidal convolution, which consists of two tasks: A distortion recognition task and a quality regression task. For the first task, image distortion type is obtained by the fully connected layer. For the second task, the image quality score is predicted during the distortion recognition progress. Experimental results on three famous IQA datasets show that the proposed method has better performance than the previous traditional algorithms for quality prediction and distortion recognition.Keywords
Cite This Article
Citations
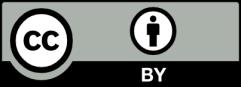
This work is licensed under a Creative Commons Attribution 4.0 International License , which permits unrestricted use, distribution, and reproduction in any medium, provided the original work is properly cited.