Open Access
ARTICLE
A Privacy Preserving Deep Linear Regression Scheme Based on Homomorphic Encryption
1 School of Computer and Software, Nanjing University of Information Science and Technology, Nanjing, 210044, China.
* Corresponding Author: Danping Dong. Email: .
Journal on Big Data 2019, 1(3), 145-150. https://doi.org/10.32604/jbd.2019.08706
Abstract
This paper proposes a strategy for machine learning in the ciphertext domain. The data to be trained in the linear regression equation is encrypted by SHE homomorphic encryption, and then trained in the ciphertext domain. At the same time, it is guaranteed that the error of the training results between the ciphertext domain and the plaintext domain is in a controllable range. After the training, the ciphertext can be decrypted and restored to the original plaintext training data.Keywords
Cite This Article
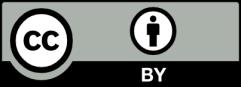
This work is licensed under a Creative Commons Attribution 4.0 International License , which permits unrestricted use, distribution, and reproduction in any medium, provided the original work is properly cited.