Open Access
ARTICLE
Multi-Scale Variation Prediction of PM2.5 Concentration Based on a Monte Carlo Method
School of Mathematics and Statistics, Nanjing University of Information Science and Technology, Nanjing, 210044, China.
School of Computing, Edinburgh Napier University, Edinburgh, UK.
*Corresponding Author: Guizhi Wang. Email: .
Journal on Big Data 2019, 1(2), 55-69. https://doi.org/10.32604/jbd.2019.06110
Abstract
Haze concentration prediction, especially PM2.5, has always been a significant focus of air quality research, which is necessary to start a deep study. Aimed at predicting the monthly average concentration of PM2.5 in Beijing, a novel method based on Monte Carlo model is conducted. In order to fully exploit the value of PM2.5 data, we take logarithmic processing of the original PM2.5 data and propose two different scales of the daily concentration and the daily chain development speed of PM2.5 respectively. The results show that these data are both approximately normal distribution. On the basis of the results, a Monte Carlo method can be applied to establish a probability model of normal distribution based on two different variables and random sampling numbers can also be generated by computer. Through a large number of simulation experiments, the average monthly concentration of PM2.5 in Beijing and the general trend of PM2.5 can be obtained. By comparing the errors between the real data and the predicted data, the Monte Carlo method is reliable in predicting the PM2.5 monthly mean concentration in the area. This study also provides a feasible method that may be applied in other studies to predict other pollutants with large scale time series dataKeywords
Cite This Article
Citations
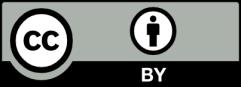
This work is licensed under a Creative Commons Attribution 4.0 International License , which permits unrestricted use, distribution, and reproduction in any medium, provided the original work is properly cited.