Open Access
ARTICLE
A Knowledge-Enhanced Disease Diagnosis Method Based on Prompt Learning and BERT Integration
School of Computer and Information Engineering, Shanghai Polytechnic University, Shanghai, 201209, China
* Corresponding Author: Hengyang Wu. Email:
Journal on Artificial Intelligence 2025, 7, 17-37. https://doi.org/10.32604/jai.2025.059607
Received 12 October 2024; Accepted 26 December 2024; Issue published 19 March 2025
Abstract
This paper proposes a knowledge-enhanced disease diagnosis method based on a prompt learning framework. Addressing challenges such as the complexity of medical terminology, the difficulty of constructing medical knowledge graphs, and the scarcity of medical data, the method retrieves structured knowledge from clinical cases via external knowledge graphs. The method retrieves structured knowledge from external knowledge graphs related to clinical cases, encodes it, and injects it into the prompt templates to enhance the language model’s understanding and reasoning capabilities for the task. We conducted experiments on three public datasets: CHIP-CTC, IMCS-V2-NER, and KUAKE-QTR. The results indicate that the proposed method significantly outperforms existing models across multiple evaluation metrics. Additionally, ablation studies confirmed the critical role of the knowledge injection module, as the removal of this module resulted in a significant drop in F1 score. The experimental results demonstrate that the proposed method not only effectively improves the accuracy of disease diagnosis but also enhances the interpretability of the predictions, providing more reliable support and evidence for clinical diagnosis.Keywords
Cite This Article
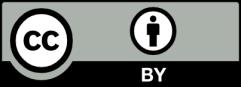
This work is licensed under a Creative Commons Attribution 4.0 International License , which permits unrestricted use, distribution, and reproduction in any medium, provided the original work is properly cited.