Open Access
ARTICLE
Pressure Classification Analysis on CNN-Transformer-LSTM Hybrid Model
1 School of Electrical and Electronic Engineering, Wuhan Polytechnic University, Wuhan, 430023, China
2 School of Mathematics and Computer Science, Wuhan Polytechnic University, Wuhan, 430048, China
3 School of Electromechanical and Intelligent Manufacturing, Huanggang Normal University, Huanggang, 438000, China
* Corresponding Author: Wu Zeng. Email:
Journal on Artificial Intelligence 2024, 6, 361-377. https://doi.org/10.32604/jai.2024.059114
Received 28 September 2024; Accepted 19 November 2024; Issue published 13 December 2024
Abstract
Stress is defined as a subjective reflection of an internal psychological state of tension or arousal, manifesting as an interpretive, emotional, and defensive coping process within the body. Prolonged and sustained stress can significantly increase the risk of psychological and physiological disorders. Heart rate variability (HRV) is a key biomarker for assessing autonomic cardiac function, typically increasing during relaxation and decreasing under stress. Although measuring stress through physiological parameters like HRV is a common approach, achieving ultra-high accuracy based on HRV measurements remains a challenging task. In this study, the role of HRV features as biomarkers for stress detection was investigated, leading to the development of an advanced CNN-Transformer-LSTM multi-class stress detection model that leverages both the time-domain and frequency-domain characteristics of HRV. Specifically, the model incorporates a convolutional neural network (CNN) combined with a classifier that integrates long short-term memory (LSTM) networks and a Transformer architecture. The CNN effectively captures local patterns and features within time-series data, while the LSTM networks manage temporal dependencies. The Transformer component enhances the model’s ability to understand the relationships between different time points in the HRV signal through self-attention mechanisms. HRV signals were extracted from the SWELL-KW database and cross-validated using the WESAD database. Experimental results demonstrate that the proposed method achieves higher accuracy compared to existing studies in the literature, even when using fewer HRV features. The obtained stress detection accuracy ranged between 98.84% and 99.31%. Additionally, this study employed marginal utility analysis to validate the effectiveness of HRV features in stress detection.Keywords
Cite This Article
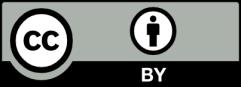
This work is licensed under a Creative Commons Attribution 4.0 International License , which permits unrestricted use, distribution, and reproduction in any medium, provided the original work is properly cited.