Open Access
ARTICLE
A Hybrid Query-Based Extractive Text Summarization Based on K-Means and Latent Dirichlet Allocation Techniques
1 Department of Computer Science, City University of Science and IT, Peshawar, 24370, Pakistan
2 Department of Computer and Software Technology, University of Swat, Swat, 19120, Pakistan
3 Department of Computer Science, IQRA National University Swat, Swat, 19130, Pakistan
* Corresponding Author: Sarwar Shah Khan. Email:
Journal on Artificial Intelligence 2024, 6, 193-209. https://doi.org/10.32604/jai.2024.052099
Received 22 March 2024; Accepted 08 July 2024; Issue published 07 August 2024
Abstract
Retrieving information from evolving digital data collection using a user’s query is always essential and needs efficient retrieval mechanisms that help reduce the required time from such massive collections. Large-scale time consumption is certain to scan and analyze to retrieve the most relevant textual data item from all the documents required a sophisticated technique for a query against the document collection. It is always challenging to retrieve a more accurate and fast retrieval from a large collection. Text summarization is a dominant research field in information retrieval and text processing to locate the most appropriate data object as single or multiple documents from the collection. Machine learning and knowledge-based techniques are the two query-based extractive text summarization techniques in Natural Language Processing (NLP) which can be used for precise retrieval and are considered to be the best option. NLP uses machine learning approaches for both supervised and unsupervised learning for calculating probabilistic features. The study aims to propose a hybrid approach for query-based extractive text summarization in the research study. Text-Rank Algorithm is used as a core algorithm for the flow of an implementation of the approach to gain the required goals. Query-based text summarization of multiple documents using a hybrid approach, combining the K-Means clustering technique with Latent Dirichlet Allocation (LDA) as topic modeling technique produces 0.288, 0.631, and 0.328 for precision, recall, and F-score, respectively. The results show that the proposed hybrid approach performs better than the graph-based independent approach and the sentences and word frequency-based approach.Keywords
Cite This Article
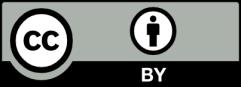
This work is licensed under a Creative Commons Attribution 4.0 International License , which permits unrestricted use, distribution, and reproduction in any medium, provided the original work is properly cited.