Open Access
ARTICLE
Enhancing Exam Preparation through Topic Modelling and Key Topic Identification
School of Computer Engineering, Kalinga Institute of Industrial Technology, Bhubaneswar, 751024, India
* Corresponding Author: Rudraneel Dutta. Email:
Journal on Artificial Intelligence 2024, 6, 177-192. https://doi.org/10.32604/jai.2024.050706
Received 14 February 2024; Accepted 17 June 2024; Issue published 19 July 2024
Abstract
Traditionally, exam preparation involves manually analyzing past question papers to identify and prioritize key topics. This research proposes a data-driven solution to automate this process using techniques like Document Layout Segmentation, Optical Character Recognition (OCR), and Latent Dirichlet Allocation (LDA) for topic modelling. This study aims to develop a system that utilizes machine learning and topic modelling to identify and rank key topics from historical exam papers, aiding students in efficient exam preparation. The research addresses the difficulty in exam preparation due to the manual and labour-intensive process of analyzing past exam papers to identify and prioritize key topics. This approach is designed to streamline and optimize exam preparation, making it easier for students to focus on the most relevant topics, thereby using their efforts more effectively. The process involves three stages: (i) Document Layout Segmentation and Data Preparation, using deep learning techniques to separate text from non-textual content in past exam papers, (ii) Text Extraction and Processing using OCR to convert images into machine-readable text, and (iii) Topic Modeling with LDA to identify key topics covered in the exams. The research demonstrates the effectiveness of the proposed method in identifying and prioritizing key topics from exam papers. The LDA model successfully extracts relevant themes, aiding students in focusing their study efforts. The research presents a promising approach for optimizing exam preparation. By leveraging machine learning and topic modelling, the system offers a data-driven and efficient solution for students to prioritize their study efforts. Future work includes expanding the dataset size to further enhance model accuracy. Additionally, integration with educational platforms holds potential for personalized recommendations and adaptive learning experiences.Keywords
Cite This Article
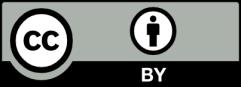