Open Access
ARTICLE
Deep Learning: A Theoretical Framework with Applications in Cyberattack Detection
Department of Electrical Engineering and Computer Science, Alabama A&M University, Huntsville, AL 35803, USA
* Corresponding Author: Kaveh Heidary. Email:
Journal on Artificial Intelligence 2024, 6, 153-175. https://doi.org/10.32604/jai.2024.050563
Received 10 February 2024; Accepted 28 May 2024; Issue published 18 July 2024
Abstract
This paper provides a detailed mathematical model governing the operation of feedforward neural networks (FFNN) and derives the backpropagation formulation utilized in the training process. Network protection systems must ensure secure access to the Internet, reliability of network services, consistency of applications, safeguarding of stored information, and data integrity while in transit across networks. The paper reports on the application of neural networks (NN) and deep learning (DL) analytics to the detection of network traffic anomalies, including network intrusions, and the timely prevention and mitigation of cyberattacks. Among the most prevalent cyber threats are R2L, U2L, probe, and distributed denial of service (DDoS), which disrupt normal network operations and interrupt vital services. Robust detection of the early stage of cyberattack phenomena and the consistent blockade of attack traffic including DDoS network packets comprise preventive measures that constitute effective means for cyber defense. The proposed system is an NN that utilizes a set of thirty-eight packet features for the real-time binary classification of network traffic. The NN system is trained with a dataset containing the packet attributes of a mix of normal and attack traffic. In this study, the KDD99 dataset, which was prepared by the MIT Lincoln Lab for the 1998 DARPA Intrusion Detection Evaluation Program, was used to train the NN and test its performance. It has been shown that an NN comprised of one or two hidden layers, with each layer containing a few neural nodes, can be trained to detect attack packets with concurrently high precision and recall.Keywords
Cite This Article
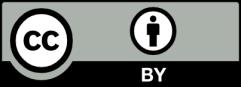
This work is licensed under a Creative Commons Attribution 4.0 International License , which permits unrestricted use, distribution, and reproduction in any medium, provided the original work is properly cited.