Open Access
ARTICLE
Cardiovascular Disease Prediction Using Risk Factors: A Comparative Performance Analysis of Machine Learning Models
1 School of Electronics and Control Engineering, Chang’an University, Xi’an, 710000, China
2 School of Information Engineering, Chang’an University, Xi’an, 710000, China
* Corresponding Author: Adil Hussain. Email:
Journal on Artificial Intelligence 2024, 6, 129-152. https://doi.org/10.32604/jai.2024.050277
Received 01 February 2024; Accepted 01 April 2024; Issue published 21 May 2024
Abstract
The diagnosis and prognosis of cardiovascular diseases are critical medical responsibilities that assist cardiologists in correctly classifying patients and treating them accordingly. The utilization of machine learning in the medical domain has witnessed a notable surge due to its ability to discern patterns from vast amounts of data. Machine learning algorithms that can categorize cases of cardiovascular illness may help doctors reduce the number of wrong diagnoses. This research investigates the efficacy of different machine learning algorithms in predicting cardiovascular disease in accordance with risk factors. This study utilizes a variety of machine learning models, including Logistic Regression, Random Forest, Decision Tree, Extra Trees classifier, Support Vector Machine (SVM), XGBoost (XGB), Light Gradient Boosting Machine (LGBM), GaussianNB, and Multilayer Perceptron (MLP). The machine learning models are applied to a concrete dataset acquired from Kaggle. The models underwent training using a dataset that was partitioned into an 80:20 ratio. Machine learning model evaluation involves the utilization of performance measurements such as Accuracy, Precision, Recall, and ROC curves. An exhaustive evaluation is carried out to gauge the efficacy of the models.Keywords
Cite This Article
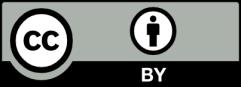
This work is licensed under a Creative Commons Attribution 4.0 International License , which permits unrestricted use, distribution, and reproduction in any medium, provided the original work is properly cited.