Open Access
ARTICLE
Detection of Student Engagement in E-Learning Environments Using EfficientnetV2-L Together with RNN-Based Models
1 Faculty of Computer Science and Information Technology, University Putra Malaysia (UPM), Serdang, Malaysia
2 Department of Electrical and Computer Engineering, University of Wisconsin, Madison, USA
* Corresponding Author: Farhad Mortezapour Shiri. Email:
Journal on Artificial Intelligence 2024, 6, 85-103. https://doi.org/10.32604/jai.2024.048911
Received 21 December 2023; Accepted 04 March 2024; Issue published 24 April 2024
Abstract
Automatic detection of student engagement levels from videos, which is a spatio-temporal classification problem is crucial for enhancing the quality of online education. This paper addresses this challenge by proposing four novel hybrid end-to-end deep learning models designed for the automatic detection of student engagement levels in e-learning videos. The evaluation of these models utilizes the DAiSEE dataset, a public repository capturing student affective states in e-learning scenarios. The initial model integrates EfficientNetV2-L with Gated Recurrent Unit (GRU) and attains an accuracy of 61.45%. Subsequently, the second model combines EfficientNetV2-L with bidirectional GRU (Bi-GRU), yielding an accuracy of 61.56%. The third and fourth models leverage a fusion of EfficientNetV2-L with Long Short-Term Memory (LSTM) and bidirectional LSTM (Bi-LSTM), achieving accuracies of 62.11% and 61.67%, respectively. Our findings demonstrate the viability of these models in effectively discerning student engagement levels, with the EfficientNetV2-L+LSTM model emerging as the most proficient, reaching an accuracy of 62.11%. This study underscores the potential of hybrid spatio-temporal networks in automating the detection of student engagement, thereby contributing to advancements in online education quality.Keywords
Cite This Article
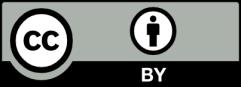
This work is licensed under a Creative Commons Attribution 4.0 International License , which permits unrestricted use, distribution, and reproduction in any medium, provided the original work is properly cited.