Open Access
ARTICLE
A Deep Learning Model for Insurance Claims Predictions
Department of Computer and Information Science, University of Strathclyde, Glasgow, UK
* Corresponding Authors: Umar Isa Abdulkadir. Email: ; Anil Fernando. Email:
Journal on Artificial Intelligence 2024, 6, 71-83. https://doi.org/10.32604/jai.2024.045332
Received 24 August 2023; Accepted 11 March 2024; Issue published 22 April 2024
Abstract
One of the significant issues the insurance industry faces is its ability to predict future claims related to individual policyholders. As risk varies from one policyholder to another, the industry has faced the challenge of using various risk factors to accurately predict the likelihood of claims by policyholders using historical data. Traditional machine-learning models that use neural networks are recognized as exceptional algorithms with predictive capabilities. This study aims to develop a deep learning model using sequential deep regression techniques for insurance claim prediction using historical data obtained from Kaggle with 1339 cases and eight variables. This study adopted a sequential model in Keras and compared the model with ReLU and Swish as activation functions. The performance metrics used during the training to evaluate the model performance are R2 score, mean square error and mean percentage error with values of 0.5%, 1.17% and 23.5%, respectively obtained using ReLU, while 0.7%, 0.82%, and 21.3%, obtained using Swish function. Although the results of both models using ReLU and Swish were fairly tolerable, the performance metrics obtained of the model using Swish, as the activation function tends to perform more satisfactorily than that of ReLU. However, to investigate the model performance deeply, therefore, this study recommends that more interest be channeled to the interpretability and explainability of the proposed model and the provisions of AI technologies by insurance industries to enhance accurate claim prediction and minimize losses.Keywords
Cite This Article
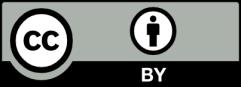
This work is licensed under a Creative Commons Attribution 4.0 International License , which permits unrestricted use, distribution, and reproduction in any medium, provided the original work is properly cited.