Open Access
ARTICLE
A Performance Analysis of Machine Learning Techniques for Credit Card Fraud Detection
1 School of Information Engineering, Chang’an University, Xi'an, 710000, China
2 School of Electronics and Control Engineering, Chang’an University, Xi'an, 710000, China
* Corresponding Author: Adil Hussain. Email:
Journal on Artificial Intelligence 2024, 6, 1-21. https://doi.org/10.32604/jai.2024.047226
Received 30 October 2023; Accepted 06 December 2023; Issue published 31 January 2024
Abstract
With the increased accessibility of global trade information, transaction fraud has become a major worry in global banking and commerce security. The incidence and magnitude of transaction fraud are increasing daily, resulting in significant financial losses for both customers and financial professionals. With improvements in data mining and machine learning in computer science, the capacity to detect transaction fraud is becoming increasingly attainable. The primary goal of this research is to undertake a comparative examination of cutting-edge machine-learning algorithms developed to detect credit card fraud. The research looks at the efficacy of these machine learning algorithms using a publicly available dataset of credit card transactions performed by European cardholders in 2023, comprising around 550,000 records. The study uses this dataset to assess the performance of well-established machine learning models, measuring their accuracy, recall, and F1 score. In addition, the study includes a confusion matrix for all models to aid in evaluation and training time duration. Machin learning models, including Logistic regression, random forest, extra trees, and LGBM, achieve high accuracy and precision in the credit card fraud detection dataset, with a reported accuracy, recall, and F1 score of 1.00 for both classes.Keywords
Cite This Article
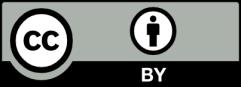
This work is licensed under a Creative Commons Attribution 4.0 International License , which permits unrestricted use, distribution, and reproduction in any medium, provided the original work is properly cited.