Open Access
ARTICLE
An Example of a Supporting Combination by Using GA to Evolve More Advanced and Deeper CNN Architecture
School of Computer and Software, Nanjing University of Information Science & Technology, Nanjing, 210044, China
* Corresponding Author: Bah Mamoudou. Email:
Journal on Artificial Intelligence 2023, 5, 163-180. https://doi.org/10.32604/jai.2023.045324
Received 23 August 2023; Accepted 23 November 2023; Issue published 26 December 2023
Abstract
It has become an annual tradition for Convolutional Neural Networks (CNNs) to continuously improve their performance in image classification and other applications. These advancements are often attributed to the adoption of more intricate network architectures, such as modules and skip connections, as well as the practice of stacking additional layers to create increasingly complex networks. However, the quest to identify the most optimized model is a daunting task, given that state of the art Convolutional Neural Network (CNN) models are manually engineered. In this research paper, we leveraged a conventional Genetic Algorithm (GA) to craft optimized Convolutional Neural Network (CNN) architectures and pinpoint the ideal set of hyper parameters for image classification tasks using the MNIST dataset. Our experimentation with the MNIST dataset yielded remarkable results. Compared to earlier semi-automatic and automated approaches, our proposed GA demonstrated its efficiency by swiftly identifying the perfect CNN design, accomplishing this feat in just 6 GPU days while achieving an outstanding accuracy of 95.50%.Keywords
Cite This Article
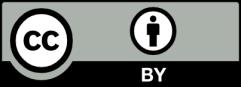