Open Access
ARTICLE
K-Hyperparameter Tuning in High-Dimensional Space Clustering: Solving Smooth Elbow Challenges Using an Ensemble Based Technique of a Self-Adapting Autoencoder and Internal Validation Indexes
1 Department of Computer Science, Riara University, Nairobi, 00200, Kenya
2 Department of Computing, Khoury College of Computer Sciences, Boston, 02115, USA
3 Department of Engineering, Kenyatta University, Nairobi, 00200, Kenya
* Corresponding Author: Rufus Gikera. Email:
Journal on Artificial Intelligence 2023, 5, 75-112. https://doi.org/10.32604/jai.2023.043229
Received 26 June 2023; Accepted 01 September 2023; Issue published 26 October 2023
Abstract
k-means is a popular clustering algorithm because of its simplicity and scalability to handle large datasets. However, one of its setbacks is the challenge of identifying the correct k-hyperparameter value. Tuning this value correctly is critical for building effective k-means models. The use of the traditional elbow method to help identify this value has a long-standing literature. However, when using this method with certain datasets, smooth curves may appear, making it challenging to identify the k-value due to its unclear nature. On the other hand, various internal validation indexes, which are proposed as a solution to this issue, may be inconsistent. Although various techniques for solving smooth elbow challenges exist, k-hyperparameter tuning in high-dimensional spaces still remains intractable and an open research issue. In this paper, we have first reviewed the existing techniques for solving smooth elbow challenges. The identified research gaps are then utilized in the development of the new technique. The new technique, referred to as the ensemble-based technique of a self-adapting autoencoder and internal validation indexes, is then validated in high-dimensional space clustering. The optimal k-value, tuned by this technique using a voting scheme, is a trade-off between the number of clusters visualized in the autoencoder’s latent space, k-value from the ensemble internal validation index score and one that generates a value of 0 or close to 0 on the derivative , at the elbow. Experimental results based on the Cochran’s Q test, ANOVA, and McNemar’s score indicate a relatively good performance of the newly developed technique in k-hyperparameter tuning.Keywords
Cite This Article
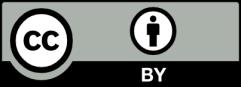
This work is licensed under a Creative Commons Attribution 4.0 International License , which permits unrestricted use, distribution, and reproduction in any medium, provided the original work is properly cited.