Open Access
ARTICLE
Implementation of Strangely Behaving Intelligent Agents to Determine Human Intervention During Reinforcement Learning
Department of Electrical & Computer Engineering and Computer Science, Jackson State University (JSU), Jackson, 39217, MS, USA
* Corresponding Author: Khalid H. Abed. Email:
Journal on Artificial Intelligence 2022, 4(4), 261-277. https://doi.org/10.32604/jai.2022.039703
Received 12 February 2023; Accepted 21 March 2023; Issue published 25 May 2023
Abstract
Intrinsic motivation helps autonomous exploring agents traverse a larger portion of their environments. However, simulations of different learning environments in previous research show that after millions of timesteps of successful training, an intrinsically motivated agent may learn to act in ways unintended by the designer. This potential for unintended actions of autonomous exploring agents poses threats to the environment and humans if operated in the real world. We investigated this topic by using Unity’s Machine Learning Agent Toolkit (ML-Agents) implementation of the Proximal Policy Optimization (PPO) algorithm with the Intrinsic Curiosity Module (ICM) to train autonomous exploring agents in three learning environments. We demonstrate that ICM, although designed to assist agent navigation in environments with sparse reward generation, increasing gradually as a tool for purposely training misbehaving agent in significantly less than 1 million timesteps. We present the following achievements: 1) experiments designed to cause agents to act undesirably, 2) a metric for gauging how well an agent achieves its goal without collisions, and 3) validation of PPO best practices. Then, we used optimized methods to improve the agent’s performance and reduce collisions within the same environments. These achievements help further our understanding of the significance of monitoring training statistics during reinforcement learning for determining how humans can intervene to improve agent safety and performance.Keywords
Cite This Article
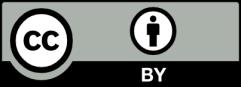