Open Access
ARTICLE
Modeling & Evaluating the Performance of Convolutional Neural Networks for Classifying Steel Surface Defects
1 Department of Computer Science, Superior University, Lahore, Pakistan
2 Department of Computer Science, University of Central Punjab, Lahore, Pakistan
3 Department of Mechanical System Engineering, Tongmyong University, Busan, Korea
* Corresponding Author: Nadeem Jabbar Chaudhry. Email:
Journal on Artificial Intelligence 2022, 4(4), 245-259. https://doi.org/10.32604/jai.2022.038875
Received 01 January 2023; Accepted 07 April 2023; Issue published 25 May 2023
Abstract
Recently, outstanding identification rates in image classification tasks were achieved by convolutional neural networks (CNNs). to use such skills, selective CNNs trained on a dataset of well-known images of metal surface defects captured with an RGB camera. Defects must be detected early to take timely corrective action due to production concerns. For image classification up till now, a model-based method has been utilized, which indicated the predicted reflection characteristics of surface defects in comparison to flaw-free surfaces. The problem of detecting steel surface defects has grown in importance as a result of the vast range of steel applications in end-product sectors such as automobiles, households, construction, etc. The manual processes for detections are time-consuming, labor-intensive, and expensive. Different strategies have been used to automate manual processes, but CNN models have proven to be the most effective rather than image processing and machine learning techniques. By using different CNN models with fine-tuning, easily compare their performance and select the best-performing model for the same kinds of tasks. However, it is important that using different CNN models either from fine tuning can be computationally expensive and time-consuming. Therefore, our study helps the upcoming researchers to choose the CNN without considering the issues of model complexity, performance, and computational resources. In this article, the performance of various CNN models like Visual Geometry Group, VGG16, VGG19, ResNet152, ResNet152V2, Xception, InceptionV3, InceptionResNetV2, NASNetLarge, MobileNetV2, and DenseNet201 with transfer learning techniques are evaluated. These models were chosen based on their popularity and impact in the field of computer vision research, as well as their performance on benchmark datasets. According to the outcomes, DenseNet201 outperformed the other CNN models and had the greatest detection rate on the NEU dataset, falling in at 98.37 percent.Keywords
Cite This Article
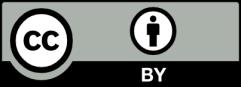
This work is licensed under a Creative Commons Attribution 4.0 International License , which permits unrestricted use, distribution, and reproduction in any medium, provided the original work is properly cited.