Open Access
ARTICLE
X-ray Based COVID-19 Classification Using Lightweight EfficientNet
Department of Computer Science, King Saud University, Riyadh, 11543, Saudi Arabia
* Corresponding Author: Tahani Maazi Almutairi. Email:
Journal on Artificial Intelligence 2022, 4(3), 167-187. https://doi.org/10.32604/jai.2022.032974
Received 03 June 2022; Accepted 19 July 2022; Issue published 01 December 2022
Abstract
The world has been suffering from the Coronavirus (COVID-19) pandemic since its appearance in late 2019. COVID-19 spread has led to a drastic increase of the number of infected people and deaths worldwide. Imminent and accurate diagnosis of positive cases emerged as a natural alternative to reduce the number of serious infections and limit the spread of the disease. In this paper, we proposed an X-ray based COVID-19 classification system that aims at diagnosing positive COVID-19 cases. Specifically, we adapted lightweight versions of EfficientNet as backbone of the proposed recognition system. Particularly, lightweight EfficientNet networks were used to build classification models able to discriminate between positive and negative COVID-19 cases using chest X-ray images. The proposed models ensure a trade-off between scaling down the architecture of the deep network to reduce the computational cost and optimizing the classification performance. In the experiments, a public dataset containing 7,345 chest X-ray images was used to train, validate and test the proposed models for binary and multi-class classification problems, respectively. The obtained results showed the EfficientNet-elite-B9-V2, which is the lightest proposed model yielded an accuracy of 96%. On the other hand, EfficientNet-lite-B0 overtook the other models, and achieved an accuracy of 99%.Keywords
Cite This Article
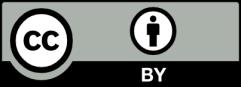
This work is licensed under a Creative Commons Attribution 4.0 International License , which permits unrestricted use, distribution, and reproduction in any medium, provided the original work is properly cited.