Open Access
REVIEW
Deep Learning-Based 3D Instance and Semantic Segmentation: A Review
1 Department of Robot System Engineering, Tongmyong University, Busan, 48520, Korea
2 School of Artificial Intelligence, Tongmyong University, Busan, 48520, Korea
* Corresponding Author: Hyunsik Ahn. Email:
Journal on Artificial Intelligence 2022, 4(2), 99-114. https://doi.org/10.32604/jai.2022.031235
Received 13 April 2022; Accepted 15 May 2022; Issue published 18 July 2022
Abstract
The process of segmenting point cloud data into several homogeneous areas with points in the same region having the same attributes is known as 3D segmentation. Segmentation is challenging with point cloud data due to substantial redundancy, fluctuating sample density and lack of apparent organization. The research area has a wide range of robotics applications, including intelligent vehicles, autonomous mapping and navigation. A number of researchers have introduced various methodologies and algorithms. Deep learning has been successfully used to a spectrum of 2D vision domains as a prevailing A.I. methods. However, due to the specific problems of processing point clouds with deep neural networks, deep learning on point clouds is still in its initial stages. This study examines many strategies that have been presented to 3D instance and semantic segmentation and gives a complete assessment of current developments in deep learning-based 3D segmentation. In these approaches’ benefits, draw backs, and design mechanisms are studied and addressed. This study evaluates the impact of various segmentation algorithms on competitiveness on various publicly accessible datasets, as well as the most often used pipelines, their advantages and limits, insightful findings and intriguing future research directions.Keywords
Cite This Article
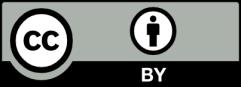