Open Access
ARTICLE
Comparative Analysis Using Machine Learning Techniques for Fine Grain Sentiments
1 Department of Computer Science, University of Gujrat, Gujrat, 50700, Pakistan
2 Faculty of Computing and Informatics, University Malaysia Sabah, Jalan UMS, Kota Kinabalu, 88400, Sabah, Malaysia
3 Department of Computer Science and Engineering, Hanyang University, Seongdong-gu, Seoul, 04763, South Korea
4 Faculty of Computing & Informatics, Multimedia University, Cyberjaya, 63100, Selangor, Malaysia
5 Department Computer Science and Technology, Chang'an University, Xi'an, 710064, China
* Corresponding Author: Kashif Nisar. Email:
Journal on Artificial Intelligence 2022, 4(1), 49-60. https://doi.org/10.32604/jai.2022.017992
Received 21 February 2021; Accepted 17 May 2021; Issue published 16 May 2022
Abstract
Huge amount of data is being produced every second for microblogs, different content sharing sites, and social networking. Sentimental classification is a tool that is frequently used to identify underlying opinions and sentiments present in the text and classifying them. It is widely used for social media platforms to find user's sentiments about a particular topic or product. Capturing, assembling, and analyzing sentiments has been challenge for researchers. To handle these challenges, we present a comparative sentiment analysis study in which we used the fine-grained Stanford Sentiment Treebank (SST) dataset, based on 215,154 exclusive texts of different lengths that are manually labeled. We present comparative sentiment analysis to solve the fine-grained sentiment classification problem. The proposed approach takes start by pre-processing the data and then apply eight machine-learning algorithms for the sentiment classification namely Support Vector Machine (SVM), Logistic Regression (LR), Neural Networks (NN), Random Forest (RF), Decision Tree (DT), K-Nearest Neighbor (KNN), Adaboost and Naïve Bayes (NB). On the basis of results obtained the accuracy, precision, recall and F1-score were calculated to draw a comparison between the classification approaches being used.Keywords
Cite This Article
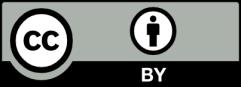
This work is licensed under a Creative Commons Attribution 4.0 International License , which permits unrestricted use, distribution, and reproduction in any medium, provided the original work is properly cited.