Open Access
ARTICLE
Solving the Feature Diversity Problem Based on Multi-Model Scheme
1 School of Telecommunication Engineering, Beijing Polytechnic, Beijing, 100176, China
2 Department of Mathematics, Wayne State University, Detroit, MI, 48202, USA
3 School of Computer Science and Technology, Tiangong University, Tianjin, 300387, China
* Corresponding Author: Jing Yu. Email:
Journal on Artificial Intelligence 2021, 3(4), 135-143. https://doi.org/10.32604/jai.2021.027154
Received 11 January 2022; Accepted 21 January 2022; Issue published 07 February 2022
Abstract
Generally, the performance of deep learning models is related to the captured features of training samples. When the training samples belong to different domains, the diverse features may increase the difficulty of training high performance models. In this paper, we built a new framework that generates multiple models on the organized samples to increase the accuracy of classification. Firstly, our framework selects some existing models and trains each of them on organized training sets to get multiple trained models. Secondly, we select some of them based on a validation set. Finally, we use some fusion method on the outputs of the selected models to get more accurate results. The experimental results show that our framework achieved higher accuracy than the existing methods. Our framework can be an option for the deep learning system to increase the classification accuracy.Keywords
Cite This Article
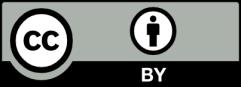
This work is licensed under a Creative Commons Attribution 4.0 International License , which permits unrestricted use, distribution, and reproduction in any medium, provided the original work is properly cited.