Open Access
ARTICLE
An Adversarial Attack System for Face Recognition
Cyberspace Institute of Advanced Technology, Guangzhou University, Guangzhou, 510006, China
* Corresponding Author: Zhaoquan Gu. Email:
Journal on Artificial Intelligence 2021, 3(1), 1-8. https://doi.org/10.32604/jai.2021.014175
Received 04 December 2020; Accepted 15 March 2021; Issue published 02 April 2021
Abstract
Deep neural networks (DNNs) are widely adopted in daily life and the security problems of DNNs have drawn attention from both scientific researchers and industrial engineers. Many related works show that DNNs are vulnerable to adversarial examples that are generated with subtle perturbation to original images in both digital domain and physical domain. As a most common application of DNNs, face recognition systems are likely to cause serious consequences if they are attacked by the adversarial examples. In this paper, we implement an adversarial attack system for face recognition in both digital domain that generates adversarial face images to fool the recognition system, and physical domain that generates customized glasses to fool the system when a person wears the glasses. Experiments show that our system attacks face recognition systems effectively. Furthermore, our system could misguide the recognition system to identify a person wearing the customized glasses as a certain target. We hope this research could help raise the attention of artificial intelligence security and promote building robust recognition systems.Keywords
Cite This Article
Citations
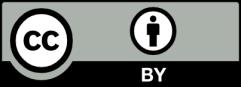