Open Access
ARTICLE
Classification Algorithm Optimization Based on Triple-GAN
1 Key Laboratory of Intelligent Computing & Information Processing, Ministry of Education, Xiangtan
University, Xiangtan, China.
2 Hunan Meteorological Information Center, Hunan Meteorological Bureau, Changsha, China.
* Corresponding Author: Jianquan Ouyang. Email: .
Journal on Artificial Intelligence 2020, 2(1), 1-15. https://doi.org/10.32604/jai.2020.09738
Received 16 January 2020; Accepted 01 April 2020; Issue published 15 July 2020
Abstract
Generating an Adversarial network (GAN) has shown great development prospects in image generation and semi-supervised learning and has evolved into TripleGAN. However, there are still two problems that need to be solved in Triple-GAN: based on the KL divergence distribution structure, gradients are easy to disappear and training instability occurs. Since Triple-GAN tags the samples manually, the manual marking workload is too large. Marked uneven and so on. This article builds on this improved Triple-GAN model (Improved Triple-GAN), which uses Random Forests to classify real samples, automate tagging of leaf nodes, and use Least Squares Generative Adversarial Networks (LSGAN) ideological structure loss function to avoid gradients disappear. Experiments were performed on the Improved Triple-GAN model and the Triple-GAN model using the MINIST, cifar10 and cifar100 datasets respectively, experiments show that the error rate of generated samples is greatly reduced. At the same time, the classification effect of the data set and the sharpness of the samples are greatly improved. And it has greatly improved the stability of model training and automation of labels.Keywords
Cite This Article
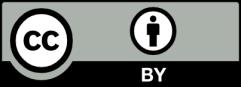
This work is licensed under a Creative Commons Attribution 4.0 International License , which permits unrestricted use, distribution, and reproduction in any medium, provided the original work is properly cited.