Open Access
ARTICLE
Remaining Useful Life Prediction of Rolling Bearings Based on Recurrent Neural Network
National Engineering Research Center of Turbo-generator Vibration, Southeast University, Nanjing, 210096, China.
School of Information Engineering, Nanjing Audit University, Nanjing, 211815, China.
Department of Mechanical and Automation Engineering, The Chinese University of Hong Kong, Shatin, Hong Kong, 999077, China
*Corresponding Author: Aidong Deng. Email: .
Journal on Artificial Intelligence 2019, 1(1), 19-27. https://doi.org/10.32604/jai.2019.05817
Abstract
In order to acquire the degradation state of rolling bearings and achieve predictive maintenance, this paper proposed a novel Remaining Useful Life (RUL) prediction of rolling bearings based on Long Short Term Memory (LSTM) neural net-work. The method is divided into two parts: feature extraction and RUL prediction. Firstly, a large number of features are extracted from the original vibration signal. After correlation analysis, the features that can better reflect the degradation trend of rolling bearings are selected as input of prediction model. In the part of RUL prediction, LSTM that making full use of the network’s memory in time is used to improve the accuracy of RUL prediction. The proposed method is validated by life cycle experimental data of bearings, and the RUL prediction results of LSTM model are compared with Support Vector Regression (SVR) and Light Gradient Boosting Machine (LightGBM) models respectively. The results show that the proposed method is more suitable for RUL prediction of rolling bearings.Keywords
Cite This Article
Citations
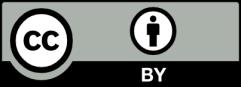
This work is licensed under a Creative Commons Attribution 4.0 International License , which permits unrestricted use, distribution, and reproduction in any medium, provided the original work is properly cited.