Open Access
ARTICLE
Underground Disease Detection Based on Cloud Computing and Attention Region Neural Network
State Key Laboratory of Coal Resources and Safe Mining, China University of Mining & Technology, Beijing, 100083, China.
School of Mechanical Electronic & Information Engineering, China University of Mining & Technology, Beijing, 100083, China.
Computer Science & Engineering, Harbin Engineering University, Harbin , 150001, China.
Computer Science & Engineering, Hong Kong University of Science and Technology, Hong Kong, China .
Stanford University, Palo Alto, CA 94305-6104, United States.
*Corresponding Author: Ce Li. Email: .
Journal on Artificial Intelligence 2019, 1(1), 9-18. https://doi.org/10.32604/jai.2019.06157
Abstract
Detecting the underground disease is very crucial for the roadbed health monitoring and maintenance of transport facilities, since it is very closely related to the structural health and reliability with the rapid development of road traffic. Ground penetrating radar (GPR) is widely used to detect road and underground diseases. However, it is still a challenging task due to data access anywhere, transmission security and data processing on cloud. Cloud computing can provide scalable and powerful technologies for large-scale storage, processing and dissemination of GPR data. Combined with cloud computing and radar detection technology, it is possible to locate the underground disease quickly and accurately. This paper deploys the framework of a ground disease detection system based on cloud computing and proposes an attention region convolution neural network for object detection in the GPR images. Experimental results of the precision and recall metrics show that the proposed approach is more efficient than traditional objection detection method in ground disease detection of cloud based system.Keywords
Cite This Article
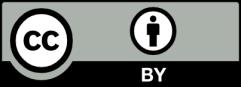