Open Access
PROCEEDINGS
Physics Informed Neural Networks (PINNs) for Multi-Step Loading in Hyperelasticity
1 Department of Mechanical Engineering, IIT Guwahati, Guwahati, 781039, Assam, India
* Corresponding Author: Sachin Singh Gautam. Email:
The International Conference on Computational & Experimental Engineering and Sciences 2024, 32(2), 1-1. https://doi.org/10.32604/icces.2024.011404
Abstract
In recent years, machine learning (ML) has emerged as a powerful tool for addressing complex problems in the realms of science and engineering. However, the effectiveness of many state-of-the-art ML techniques is hindered by the limited availability of adequate data, leading to issues of robustness and convergence. Consequently, inferences drawn from such models are often based on partial information. In a seminal contribution, Raissi et al. [1] introduced the concept of physics informed neural networks (PINNs), presenting a novel paradigm in the domain of function approximation by artificial neural networks (ANNs). This advancement marks a significant step forward in leveraging ML for solving complex problems, offering a promising avenue for enhancing the robustness and reliability of ML models in scientific and engineering applications.PINNs represent a novel approach to solving partial differential equations (PDEs) using machine learning. PINNs are particularly useful in scenarios where data is limited, as they can leverage both the available data and the underlying physics of the problem. The key idea behind PINNs is to incorporate the governing PDEs into the neural network's training process. This allows the model to learn the solution to the PDEs directly, without relying on traditional numerical solvers. By combining data-driven learning with physical principles, PINNs offer a promising avenue for solving complex PDEs efficiently and accurately.
In this study, we propose a PINN framework to tackle solid mechanics problems, with a specific focus on handling multi-step loading scenarios. While our formulation is applicable to a wide range of solid mechanics problems, we concentrate on the realm of two-dimensional hyperelasticity.
We demonstrate the efficacy of our approach through a case study involving a hyperelastic plate subjected to compression, where the displacement load is applied incrementally. To construct our loss function, we utilize the deep collocation method [2]. In order to enhance the accuracy of our model, we train it using a combination of the Adam [3] and L-BFGS optimizers.
We validate our results by comparing the displacement and Cauchy stress obtained from our PINN model with those from a standard finite element analysis (FEA). Our findings indicate that the results obtained from the PINN model are in excellent agreement with those from FEA, demonstrating the effectiveness of our approach in accurately capturing the behavior of solid mechanics systems under multi-step loading conditions.
Keywords
Cite This Article
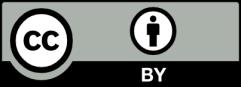
This work is licensed under a Creative Commons Attribution 4.0 International License , which permits unrestricted use, distribution, and reproduction in any medium, provided the original work is properly cited.