Open Access
PROCEEDINGS
Design of Anisotropic Heat Conduction Structures Based on Deep Learning
1 State Key Laboratory of Intelligent Manufacturing Equipment and Technology, Huazhong University of Science and Technology, Wuhan, 430074, China
* Corresponding Author: Mi Xiao. Email:
The International Conference on Computational & Experimental Engineering and Sciences 2024, 32(1), 1-1. https://doi.org/10.32604/icces.2024.012356
Abstract
Heat conduction structures are widely employed in thermal management of electronic components across aerospace, electronics, and related domains, ensuring sustained operational performance and longevity. However, conventional approaches to heat conduction structure design are encumbered by constraints on design flexibility, suboptimal thermal dissipation characteristics, and inefficiencies. Addressing these limitations, this study presents a novel approach leveraging deep learning for the design of anisotropic heat conduction structures. Initially, a pre-trained deep generative model is deployed to enable real-time generation of topologically functional cell (TFC) at the microscale. With the introduction of rotation angles of each TFC, these microstructures acquire anisotropic thermal properties, thereby expanding the design space. Subsequent optimization of TFC distribution within the macroscopic design domain facilitates the efficient design of heat conduction structures under diverse boundary conditions. Notably, leveraging four distinct thermal performance objectives—namely, minimum heat dissipation flexibility, minimum highest temperature, minimum average temperature, and minimum temperature difference—achieves comprehensive heat conduction structure designs, the simulated results validate the efficacy and superiority of the proposed method.Keywords
Cite This Article
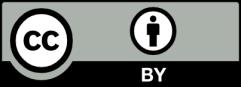
This work is licensed under a Creative Commons Attribution 4.0 International License , which permits unrestricted use, distribution, and reproduction in any medium, provided the original work is properly cited.