Open Access
PROCEEDINGS
Deep Learning Aided Optimization of 1D Phononic Crystals
1 Department of Mechanical Engineering, National Cheng Kung University, Tainan, 701, Taiwan
* Corresponding Author: I-Ling Chang. Email:
The International Conference on Computational & Experimental Engineering and Sciences 2024, 31(2), 1-1. https://doi.org/10.32604/icces.2024.012885
Abstract
In this work, a new deep learning (DL) approach for the bandgap optimization of 1-D phononic crystal will be reported. The unit cell of the phononic crystal is composed of 4 layers with 3 materials, i.e., concrete, soil and rubber. A deep learning model is trained to replace the computationally demanding traditional solvers for the bandgap calculation of 1-D phononic crystals. Four variables, including material properties and layer thicknesses, will be taken into account. The predicted bandgap by the trained model is compared with that calculated by transfer matrix in order to check the accuracy of DL model. The comparison illustrates that most data (more than 95%) is within the error lower than 1%. A genetic algorithm is adopted as the optimization tool, which combines with the trained DL model, to maximize the bandgap. It takes about 7200 seconds to optimize the design of phononic crystals with transfer matrix calculation and 2.7 seconds with DL model. Besides, the optimized bandgap difference is less than 1%, which demonstrates that the DL model could serve as a good surrogate to calculate bandgap.Keywords
Cite This Article
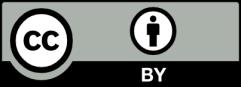
This work is licensed under a Creative Commons Attribution 4.0 International License , which permits unrestricted use, distribution, and reproduction in any medium, provided the original work is properly cited.