Open Access
PROCEEDINGS
Use of Hybrid-PINNs for Fast Predictions of Transport Structures in the Cz-Melt in Growth of Bulk Silicon Single Crystals
1 Department of Material Engineering Science, Osaka University, Machikaneyama 1-3, Toyonaka, Osaka, 560-8531, Japan
2 Department of Mechanical Engineering, University of Victoria, Victoria, BC, V8W 3P6, Canada
* Corresponding Author: Yasunori. Okano. Email:
The International Conference on Computational & Experimental Engineering and Sciences 2024, 31(2), 1-1. https://doi.org/10.32604/icces.2024.011685
Abstract
We have developed a machine learning model, called Hybrid-PINNs (Physics Informed Neural Networks), and applied for fast predictions of transport structures (flow and thermal fields) in the silicon (Si) melt during the Czochralski (Cz) bulk single crystal growth. Si bulk single crystals are mostly grown by the Cz method. For the growth of high-quality Si crystals with this method, it is essential to understand and control these transport structures in the melt. Since the direct observation of such transport fields in the melt during growth is usually impossible, numerical simulations provide a powerful tool for this purpose.Classical numerical simulations for this purpose have two major issues: a) they require long computational times and b) mesh reproduction as the melt depth decreases with the growth. Therefore, it is very difficult to carry out a real-time analysis. However, Hybrid-PINNs can make very fast predictions. For instance, they predicted the transport fields of this study in only about 0.1 second under each boundary condition while a classical numerical simulation required about 45-minute computation time. In addition, Hybrid-PINNs have a great advantage that they can provide predictions without any mesh reproduction and satisfy the governing equations. Thus, we can conclude that Hybrid-PINNs can be used as a real-time surrogate model for observations of transport structures during experiments due to their fast predictions.
Keywords
Cite This Article
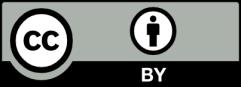
This work is licensed under a Creative Commons Attribution 4.0 International License , which permits unrestricted use, distribution, and reproduction in any medium, provided the original work is properly cited.