Open Access
PROCEEDINGS
Multi-Modality In-Situ Monitoring Big Data Mining for Enhanced Insight into the Laser Powder Bed Fusion Process, Structure, and Properties
1 Department of Mechanical Engineering and Materials Science, University of Pittsburgh, Pittsburgh, Pennsylvania 15261, USA
* Corresponding Author: Xiayun Zhao. Email:
The International Conference on Computational & Experimental Engineering and Sciences 2024, 31(2), 1-2. https://doi.org/10.32604/icces.2024.011479
Abstract
Laser powder bed fusion (LPBF) is one predominant additive manufacturing (AM) technology for producing metallic parts with sophisticated designs that can find numerous applications in critical industries such as aerospace. To achieve precise, resilient, and intelligent LPBF, a comprehensive understanding of the dynamic processes and material responses within the actual conditions of LPBF-based AM is essential. However, obtaining such insights is challenging due to the intricate interactions among the laser, powder, part layers, and gas flow, among other factors. Multimodal in-situ monitoring is desired to visualize diverse process signatures, allowing for the direct and thorough revelation of key properties. In this work, we introduce the development and application of our unique LPBF-specific integrated multi-sensor monitoring system that can effectively and simultaneously quantify multiple physics phenomena at different spatiotemporal scales. Specifically, two-wavelength imaging pyrometry is reinvented for cost-effective and data-efficient melt pool temperature and morphology monitoring with high accuracy and sufficiently fast sampling rate (> 10,000 Hz) [1, 2]. New methods of camera-based laser scan monitoring and fringe projection profilometry are developed for melt pool registration, spatter monitoring, and layer surface topography measurement [3-6]. Machine learning methods are employed to analyze and fuse the in-situ multi-monitoring data for predicting in-process and post-print part properties [3, 7, 8]. This work introduces our current monitoring systems, big data analytics methods, and exemplary application cases to predict different part properties such as solidified melt pool depth, grain length, and porosity. Experiment results show that our LPBF process monitoring systems and part characterization methods are more accurate than typical methods due to the melt pool absolute temperature measurement capability. Our work will help advance LPBF process modeling, optimization, control, and qualification. It will also facilitate the materials research such as metallurgy modeling, microstructure characterization, as well as alloy design and in-situ fabrication.Keywords
Cite This Article
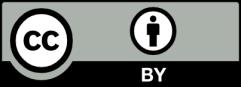
This work is licensed under a Creative Commons Attribution 4.0 International License , which permits unrestricted use, distribution, and reproduction in any medium, provided the original work is properly cited.