Open Access
PROCEEDINGS
Deep Learning-Based Prediction of Material Elastic Constants and Residual Stresses of Orthotropic Materials from Moiré Interferometry
1 Advanced Materials Research Center, Technology Innovation Institute, Abu Dhabi, 9639, UAE
2 School of Industrial Engineering, Kumoh National Institute of Technology, Gumi, 39177, South Korea
3 Civil Infrastructure and Environmental Engineering, Khalifa University of Science and Technology, Abu Dhabi, 127788, UAE
4 Department of Biomedical Engineering, Khalifa University of Science and Technology, Abu Dhabi, 127788, UAE
* Corresponding Author: Dong-Wook Lee. Email:
The International Conference on Computational & Experimental Engineering and Sciences 2024, 31(2), 1-1. https://doi.org/10.32604/icces.2024.011286
Abstract
This work analyzes the problems of material elastic constants identification and residual stresses determination in an orthotropic materials using hole drilling method. These problems are very important to understand mechanical performance of materials. A lot of optical method such as Moiré, laser speckle interferometry, digital image correlation or photoelasticity is developed to estimate displacement (or strain) fields or applied loads (or stresses) from images. These methods require a very complicated techniques, skill, and efforts to analysis images. But deep learning method based on a convolution neural network shows better performance in image analysis problems such as object classification, detection, and segmentation than the other methods and it doesn’t need human intervention which can make errors, so it can be autonomous procedure.In this work, Moiré interferometry images are generated from the displacement field around hole in a stressed orthotropic plate with plane stress condition and they are used as dataset for deep learning model. This study aims to develop a novel convolution neural network based deep learning model for inverse analysis such as material elastic constants identification and residual stresses determination in orthotropic materials and it is compared with the benchmark model such as GoogLeNet and ResNet. It has significantly fewer parameters than benchmark model, but it shows same accurate prediction of mechanical properties and residual stresses of orthotropic materials.
Keywords
Cite This Article
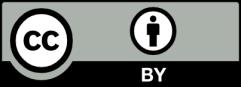
This work is licensed under a Creative Commons Attribution 4.0 International License , which permits unrestricted use, distribution, and reproduction in any medium, provided the original work is properly cited.