Open Access
PROCEEDINGS
Multi-Material Topology optimization via Stochastic Discrete Steepest Descent Multi-Valued Integer Programming
1 State Key Laboratory of Structural Analysis, Optimization and CAE Software for Industrial Equipment, International Research Center for Computational Mechanics, Department of Engineering Mechanics, Dalian University of Technology, Dalian, 116023, China
* Corresponding Author: Yuan Liang. Email:
The International Conference on Computational & Experimental Engineering and Sciences 2024, 30(4), 1-1. https://doi.org/10.32604/icces.2024.012504
Abstract
Compared to single-material optimization, topology optimization of multi-material structures offers a larger design space. It also requires efficient material selection methods to provide guidance for designers. The predominant methods are based on interpolation schemes, which introduce order-dependence issues during the optimization process. This means the sequence in which materials are arranged can significantly impact the optimization outcomes and may lead to notable issues with material gradation. This paper identifies the mathematical essence of multi-material topology optimization as a nonlinear multi-valued integer programming problem. In this paper, we propose a novel stochastic discrete steepest descent multi-valued integer programming algorithm based on the discrete convex analysis theory, which has been successfully applied to challenges in topology optimization, including compliance, frequency, and frequency constraints. Numerical examples demonstrate the effectiveness of the proposed algorithm, showing its ability to generate designs with clear material interfaces, independent of the ordering of candidate materials. This algorithm also shows promise for broader application in large-scale topology optimization problems that involve multi-valued categorical design variables.Keywords
Cite This Article
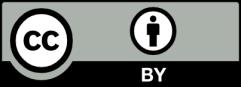
This work is licensed under a Creative Commons Attribution 4.0 International License , which permits unrestricted use, distribution, and reproduction in any medium, provided the original work is properly cited.